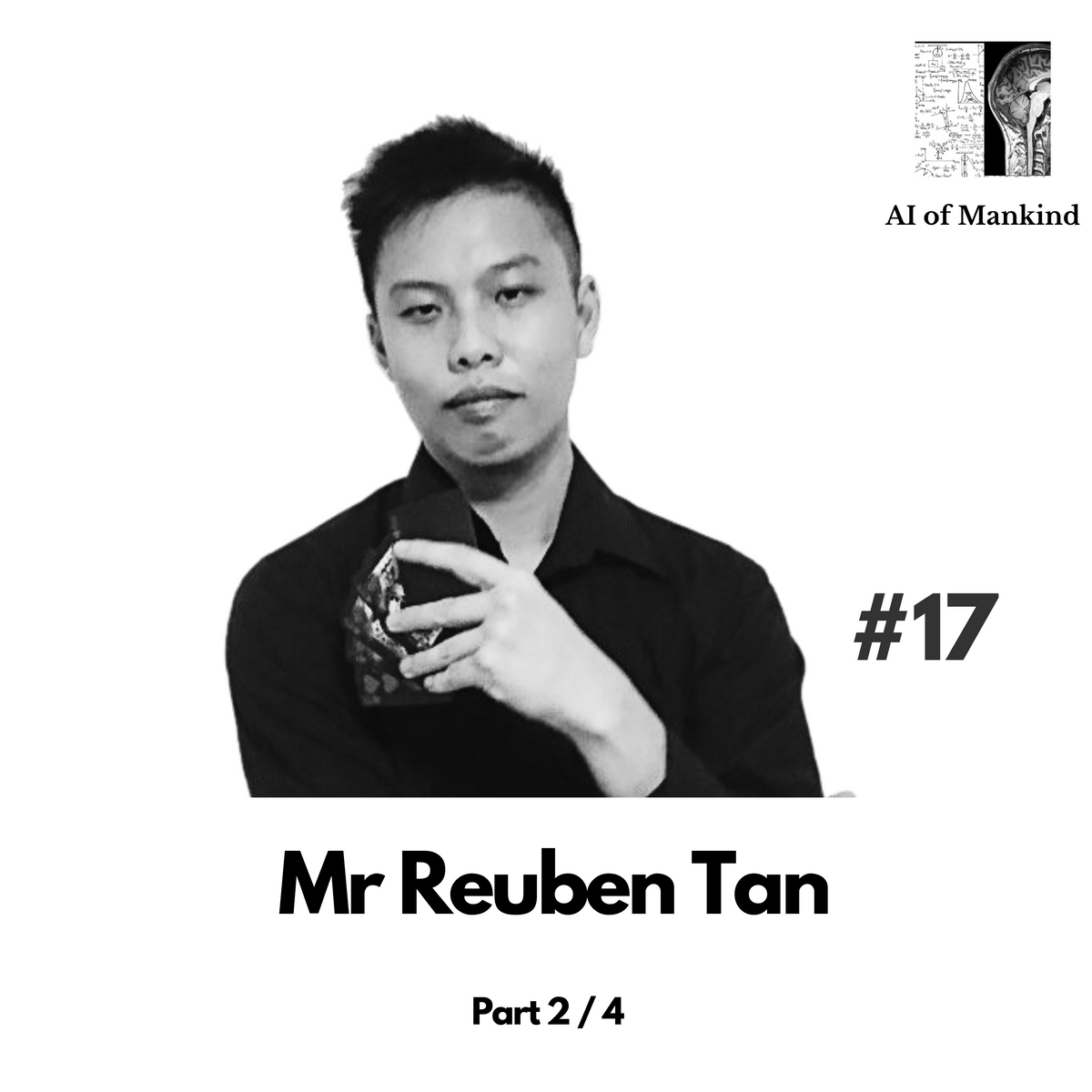
Trailer
Podcast with Reuben Tan Part 2
Podcast with Reuben Part 2
Summary:
Leadership is about taking ownership, figuring out what went wrong, offering possibilities, and getting buy-in from the stakeholders. Cost-benefit scalability analysis helps a company to evaluate the profitability and return of investment of doing digital transformation as the company scale this technological capability over more customers and over different locations. Reuben mentioned that the company can have decreasing return to scale of using AI if the people do not implement AI well. Reuben believes Elon’s musk school of thought on the future of AI is most likely the prevailing scenario and societies need to be prepared for it.
[00:00:00] Andrew Liew Weida: Hi, everyone. Welcome to the AI of mankind show where I share anything interesting about mankind. I'm your host for this season. My name is Andrew Liew. I work across four Continents and 12 international cities. Also, I work in tech startups across a range of roles from selling products, making customers happy, figuring out fundraising, making finance tick, building teams, and developing sticky products. Apart from building startups. I've also worked in fortune 500 companies as a chief data scientist or technologist or people leader. You can call me Jack of all trades or master of learning. I hope to make this podcast show a great learning experience for us In each season, there is a series of interesting things where I invite guests to share their views about their life and interests.
[00:01:09] Andrew Liew Weida: Now let the show begin.
[00:01:26] Andrew Liew: In the previous episode, Reuben talks about his backstory. Along the way, he shared a use case of choosing the right data and metrics for a digital transformation use case: a digital marketing campaign. This episode continues the part 2 conversation with Reuben and Reuben shared with us his views on leadership and cost-benefit scalability analysis. Reuben shared an example of the concept of decreasing return to scale on the application of AI. Lastly, Reuben shared his views on the future of AI and the 2 schools of thought.
[00:02:01] Andrew Liew Weida: This is what we call leadership. You step up to the role, you figure out that, Hey, this might not be the best indicator for this particular case. And you try. And if it was great, if it doesn't work, at least you try, you show that, Hey, you have done your job in your utmost best to explain why they should consider cost per action, or maybe impression, instead of click-through rate. Like it could be some other metrics that you feel that it makes better sense for the company than " the easy to game" click-through rate. We talk about digital transformation, about starting small. Most companies will tend to start small and they will start to deploy AI and digital transformation together. Because of the fact that you've done natural language programming, computer vision, regression models, predictions, and now recently campaign optimization. What is AI's role in digital transformation?
[00:02:50] Reuben Tan: In my opinion AI like any like coding and technology, it's just actually an automation tool. AI is just like the brains on how to automate stuff. And every task requires the right tool and every tool also has a cost. So you're not gonna buy a compiler just to go and skin some chicken. It's not effective. It's not feasible. So AI has amazing and almost tremendous potential, but relevancy has to be evaluated on the case by case basis. So before embarking on a project, we have to do a cost-benefit, and scalability analysis. Not every project has to be a self-driving car. That's it. We can use AI to automate simple or TDS tasks and use data science to make more driven decisions. A simple example could be looking at customer usage features of a product. Let's say you have continuous data. You have a lot more time when customers use your data. So from there. you'll be able to get their last usage and the frequency of usage. And the volume that they used be like a transaction or something, then you can, okay, so you have this recency frequency model. From there, you can start actually profiling these customers, cluster put in a specific profile. Then from there, you can look within the profile, even look across the profile. So you, start to run campaigns to upsell across sell these customers. And another step further, you can even start to estimate figures like lifetime value and the customer really attention rate reach should these customers to when to try to.
[00:04:13] Andrew Liew Weida: AI has so much potential and before embarking on any project, it is always best to do a cost-benefit, scalability analysis. And then you also talk about its automation tools and you give an example of the marketing campaign, every few segmentation or clustering. Now I wanna come back to that first point that you mentioned about Doing cost-benefit, and scalability analysis because cost-benefit analysis and any economic terms are about we want to do this initiative and what's the total cost that goes into this. And what are the benefits and do you compare the benefits minus the cost and see whether the benefits outweigh the cost? If so, then go ahead. If not, then let's either shelve it or drop it. So now you added this new term, the cost-benefit, scalability analysis. Tell us more about what does it mean to you?
[00:05:07] Reuben Tan: As we just roughly define a bit more cost-benefit analysis, normally it's if the project is a long time horizon, I say 10 years, you do some kind of discount to go and look at the future benefits. I believe that the dimension of scalability, because when it comes to technology, sometimes we have to also go and think about how scalable it is. So in a sense, it's discounting back the scalability to present time value.
[00:05:30] Andrew Liew Weida: To the audience out there, especially the business folks or the layman folks what does scalability means? For example, let's say you build a simple website, and you sell one item, and this website to our audience out there who knows technical times got monolithic, meaning it's a very simple structure. It has input-output, you click, you buy stuff, If there are 10 customers a day, maybe a simple website can do it. But if there are 1 million customers suddenly comes a day, what happened to the website? The website will crash. So if the project is not built for scalability, then if we figure out that the next initiative is not built for scalability, maybe it becomes a greater cost than the benefit.
[00:06:11] Reuben Tan: This is a very good example because sometimes it may be actually, losing your money to scale. And in fact, you have no trust, but support otherwise the company's reputation will take a hit, which is an even bigger loss. So supporting this project becomes a loss, so definitely evaluate a project's scalability as well. So in that point that you gave for the website, in that sense, you need to think, okay, now I have a million customers. How am I going to scale this? Am I going to use some kind of caching? Am I going to use some kind of data sharding, if there's time in the back? And not just maybe 1 million customers in one region. Maybe now it's the two, or three regions. Oh, how am I gonna bring all this to another region? There's a whole next level of problem. Cause you can't just think local. You have to think globally eventually if you want the company to grow bigger.
[00:06:56] Andrew Liew Weida: I just remember there was economics, there was the same term called the increasing return to scale and decreasing return to scale. In general, in most cases, AI is supposed to have an increasing return to scale because as you have more data, the AI is able to give a better more responsive, and more efficient way of deploying the resources to complete a task. Have you seen any cases where AI, if not properly constructed, could lead to decreasing return to scale?
[00:07:28] Reuben Tan: One of them is this is actually something to computer vision. So yes as we if you wanna train a new model. You first have to label the data. So in the sense of computer vision let's say, I want to have software that recognizes cats. So then we have to label the cat manually. Now let's crowdsource it. So we get 5,000 people to label a hundred images of cats. Now here comes the problem. Okay. Now, this thing has skewed a lot, there's a lot more data, but the problem is everybody labels this data differently. So let's say I have a picture of a cat. Like the cat is facing you. Is it just a cat's face? No worries. No problem. But what if is that there are three cats in the picture? Maybe person A will label all three of them as one cat. Person B will label the cat's face, the third cat face. Person C will label the cat, the first cat's whole body with the face, and the cat's whole body as the face. So now this becomes a problem.
[00:08:20] Andrew Liew Weida: This is a very common problem. I remember Google basically bought over a company called catcher. And they were trying to solve this kind of like mislabeling or MIS annotation problem because they realize that, Hey, yeah, even though we hire a bunch of people to label the cat by looking at the face or some features to label it correctly. But like you said, if there are three cats or 4 cats, maybe the guy is just being lazy. Ah. This is not a cat. This is some dirty labeling the rubbish in rubbish out you know. So without proper labeling. AI created in this context decreasing return scale. Coming back to the part about AI being [00:09:00] efficient at automating a lot of stuff, because it's able to mimic human intelligence to do repetitive stuff. There are always two schools of thought, one was by Jack ma and one was Elon Musk. Jack ma is the optimistic saying, Hey the future will be very bright. AI will create a lot of new possibilities. And Elon Musk saying. Hey AI will eventually automate almost 90% of the job and a lot of people with simple skills will not have a job. What do you think about the future of AI?
[00:09:28] Reuben Tan: I feel that AI is actually a hot field, with a lot of research going on. A lot of things less, slightly gonna be automated . One point that both of them agree on is there's gonna be a lot of automation. A lot of jobs will be taken away. That said. I'm gonna be hopeful. This is a good thing. Maybe things like UBI, universal basic income will have to really start being explored. Naturally, there are think a lot of things that can go wrong a lot, but I would believe that yeah it is the first step toward creating an equal society. So I like to imagine maybe Elon Musk is correct. Nobody has to do work, but they can still enjoy life. So [00:10:00] imagine a world where people work because they have an idea. They learn to benefit humanity or simply because they have a passion for something. So let's say we cooking. So maybe this AI automation can bring value to a chef who wants to experiment with recipes, but it's a bit easier to find the ingredients. So maybe you have a bot with RPA can go peel potatoes, dice the onions then the chef himself can assemble the stuff. If it is true, it is probably a relatively further future, a more realistic view of things in a coming couple of decades. I think more likely you will see AI slowly replacing a lot more straightforward tasks and even sure our work will probably drift to more analysis work. Mainly will be doing QA and things that cannot be done by the AI. So this is where the gap is. And like I can envision a very positive future and I can also see the future. I feel like whichever future we end up at right will be the result in the next couple of decades of how things unfold. And I hope that humanity uses AI for the better to actually benefit the common good of mankind.
[00:10:56] Andrew Liew Weida: so you share some examples of how each school is taught, and actually plays out over time. And one thing you agreed that AI really augmented or automates a lot of jobs and living behind new jobs that require creativity and a lot of analytical work. We still need doctors to do surgery, even though they're still robotic surgery, but because the surgery is so complex, the robots actually augmented the task to make it simple, more precise, and cutting and stitching up. And most patients, also wouldn't dare to take surgery via Mr. Robot.
[00:11:30] Andrew Liew: Hi everyone, thanks for tuning into this episode. We have come to the end of part 2 with Reuben. In the next episode, we will continue with Reuben in part 3 which he shared with us his views on the chicken rice hawker's future. Reuben shared his view on the beautiful future of AI and the possible need for UBI, universal benefit income. If this is the first time you are tuning in. Remember to subscribe to this show. If you have subscribed to this show and love this episode Please share it with your friends, family, and acquaintances. See you later and see you soon.