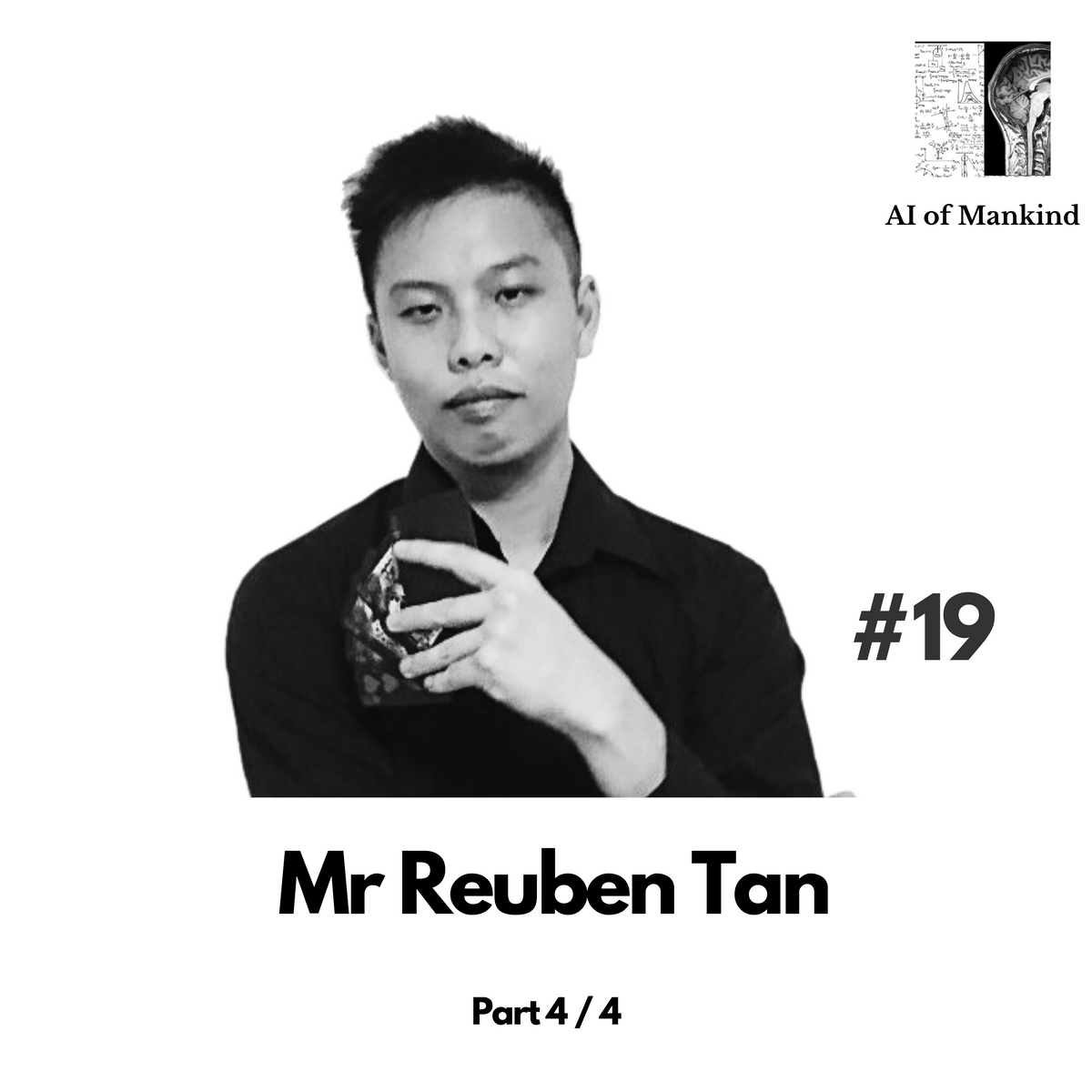
Trailer
Podcast with Reuben Tan Part 4
Podcast with Reuben Part 4
Summary:
Reuben believes that employees will not join companies that don’t invest in them. Companies need to give time and be patient in order for people to learn and translate learning to problem solving. Reuben mentioned that 2 projects per person are good rules of thumb to manage workload for projects but the timeline depends on the scale of the project. Companies that support the 80% rule are crucial to their success with talents. If a company views itself like a VC and invests in people and projects with a risk appetite, then the company might reap unbelievable rewards. Reuben also believes the BYOS (bring your own stack) is the best way to attract tech talents to get going relative to joining a company with a centralised tech doctrine in the long run. Reuben shared his thoughts on his choice of book, apps, and career advice for anyone trying to go into digital, data careers.
[00:00:00] Andrew Liew Weida: Hi, everyone. Welcome to the AI of mankind show where I share anything interesting about mankind. I'm your host for this season. My name is Andrew Liew. I work across four Continents and 12 international cities. Also, I work in tech startups across a range of roles from selling products, making customers happy, figuring out fundraising, making finance tick, building teams, and developing sticky products. Apart from building startups. I've also worked in fortune 500 companies as a chief data scientist or technologist or people leader. You can call me Jack of all trades or master of learning. I hope to make this podcast show a great learning experience for us In each season, there is a series of interesting things where I invite guests to share their views about their life and interests.
[00:01:09] Andrew Liew Weida: Now let the show begin.
[00:01:13] Andrew Liew: In the previous episode, Reuben shared his views on the chicken rice hawker's future. Reuben shared his view on the beautiful future of AI and the possible need for UBI, universal benefit income. This episode continues the part 4 conversation with Reuben and Reuben shared his career advice on how to build a career in AI , digital and data. Let's continue.
[00:01:35] Reuben Tan: And I want to point out that in the job market employers are not the only players, potential employees. So actually I feel like they would be unwilling to join a company that does not invest in them and helps them be good with their skills and the career. And this will result in a visual vicious cycle of those with skills and talent, leaving their company and making potential employees even more apprehensive about joining their place.
[00:01:57] Andrew Liew: So coming back, you mentioned these] days the younger generation of talent or employees, no longer will just look at, just pay itself, but does the company really invest in its people in terms of giving them an opportunity to learn. So talking about that and we also experienced it before there are some teams or some subculture department that they say, Hey Reuben. I support you. You go and do the learning, then you ask them, Hey this thing may be, I need to learn let's say NLP probably take me maybe three months or six months to learn and successfully complete work working project to learn like, oh sorry this doesn't work. What do you have to say about some of the managers that they pay lip service to say you go and learn, but then in 3 days, gimme a task done? What do you have to say about that?
[00:02:43] Reuben Tan: I feel like this is not great. This is not a good tech culture. I feel tech culture. You really have to be very flexible. Okay. In the tech paradigm, there's agile and waterfall. I still prefer waterfall in a sense, because it's really very hard to give estimates, especially when things are new. Maybe if you're doing something that's very established, like web development, things are very simple. I want the new picture and this thing, the page, or a very simple application then. Yeah. Then Agile really makes sense, but when it comes to exploration, and learning new things, It does not make sense to actually force your timeline because believe when it comes to learning, it's best to try. And when you try, sometimes even you are inevitably hit blockers and these blockers without proper guidance is gonna be very difficult to get past them. And it's just not realistic to accept people to solve them in a day or two. Sometimes people can get blocked for a very long time. They can put it on hold, they can just work on some other task at hand. Then they do get inspiration in the middle of somewhere, then they can attempt the problem again. So I feel that this is how it is when it comes to R and D . It is very hard to force innovation.
[00:03:49] Andrew Liew Weida: I sort of resonate with you that. I think one of the tell-tale sign of whether a company really say what they mean or put the time and effort into where they believe that they invest in training or skilling is that you look at two things. One is the number of projects they put you onto. And the time that they give you a buffer to fill and learn and continue to reach something. So like we mentioned, like 3 days to learn and come up with something in the new world and R and D whether is the AI, or creative projects. It's just ridiculously too short at times. I would say maybe three months or six months on one particular project. And also the number of projects that the individual is to work on. I feel that the rate shouldn't be more than two to three because if it's more than four or five up to six, then the guy will never be able to focus on like one or two of the hot blockers to work it out. He will be like in the Singapore term, we call it the roti prata and in the western world is called it the pancake. You spread so thin and it breaks. In your case, what is a good magic number in terms of the number of projects and the timeline for each project?
[00:05:05] Reuben Tan: I would agree if you on the number just two or three. The timeline wise it always depends on how big the scale is, but I feel like the most important thing for R and D staff, is accountability and transparency. Don't give a timeline but we would set, check-in instead.. So maybe let's say I'm working with you. I'm trying to invent something like maybe on the inventor automated Prata maker now
[00:05:26] Andrew Liew Weida: haha
[00:05:27] Reuben Tan: so maybe I just check in your view. Every couple of it. I guess I just walk through view what I did and then you're not just a manager. You are like a leader, so you say, Hey, you. Okay. You try this approach and fail. No worries. Have you tried doing this? How about this direction? So you try to give ideas like we are working together as a team, you pushing in a common direction.
[00:05:44] Reuben Tan: It's not like: that you do this. No. This is definitely a very toxic culture and I believe nobody want it. No innovation will come out of this kind of work environment.
[00:05:54] Andrew Liew Weida: Yeah.
[00:05:54] Reuben Tan: This is so why a lot of other tech companies like your FANG or rather now MANG, [00:06:00] because Facebook has changed his name. I believe they have this 80% rule, like 80% of the time is spent implementing projects. And so 20% of the time is spent just being they just trying stuff. There's a lot of room for failure and I believe this is very crucial to their success. You need room to experiment and grow and occasionally fail.
[00:06:18] Andrew Liew Weida: I really also believe in that there's such learning and investment of skilling to allow people to roam to a certain degree, like some psychological safety so that they can be more effective at getting things done. But then there are some business leaders that need to be more patient, need to be more chill because they have to think that: Hey if I spend too much money on R and D and that thing gets out the company's not making money, I'll be out of a job. I think it takes a lot of courage. Don't you think?
[00:06:46] Reuben Tan: Yes. I agree because it's always a resource. So I can understand that they are also under pressure. So I believe that's why it's best to have employees working on something that actually can make a profit. And at the same time, give them some path that [00:07:00] could also be a moonshot. And if the company views themself in a sense, like a VC, if they do this across other company, no matter we have a hundred projects, it is like 90, we just need one would become an amazing product that we can actually make money off. And I think, I believe this is how the law of large numbers works in a sensor and how VC works. So I believe this is only applicable to larger and medium companies. So if you're a small company, you're gonna take this risk. I feel like it. It's a bit more difficult because in a sense your opportunity cost is a lot higher.
[00:07:29] Andrew Liew Weida: Smaller companies, tech, startup yes. The opportunity cost of making mistakes is higher, but also they move fast because There's no baggage. Here are the assumptions. They go into uncharted territory where regulation hasn't been formed yet. So you're free to go. Let's say when a situation where 5,000 years ago, banks weren't even a term,. There was no regulation of the amount of money you need to lend, on the amount of money you need to hold, and therefore you can experiment. And so if you think from an uncharted territory where regulatory hasn't formed, startups are [00:08:00] able to tell their new people that, Hey, go ahead and try. Go ahead and fail. Because unlike a very big bank or airline where. You screw up one thing and then the regulations come after you and eventually so much constraint. What do you think about that?
[00:08:13] Reuben Tan: Oh, I personally believe right. That's the best position to be. because you just do your own thing, nobody really knows about it and you succeed. It's so mind-blowing. It just disrupts everything. I believe that's the best place to be there, but when it comes to existing companies, they do have a lot of things that need a balance. For example, sometimes there's a lot of legacy. That's gonna weigh you down and sometimes there's really no chance la. Then it's quite reasonable for a medium and large tech startup . If you are small it's a bit harder unless they're very flexible. The benefits of a small company, the work process will probably be a lot faster so you can experiment a lot more. One of the, one of the downsides of working in larger companies, there's a lot of people that specializes in stuff, which can also be a good thing because they're good at what they do. You already know what you wanna do roughly, but you dunno who the person is and you dunno which part to apply for. So sometimes things like that can slow you down.
[00:09:05] Andrew Liew Weida: Like big companies just to get data, maybe you have to go to three or four people because data governance, you have to go through cyber security, different protocols, like six, seven steps by the time it's already three weeks and your boss say, Hey, why are you still not doing any exploratory data? Oh, boss. Because I spent three weeks just to get the data. Whereas in a startup, you'll be like, oh, you need data. Do a two-factor authentication on the AWS or Microsoft or Google cloud. Bamp! Within two days you got it right. Now coming back to the learning. How do you think about learning? Because of the enormous amount of knowledge that you mentioned about companies, or even employees should do cross-sharing, what does cross-sharing or cross-learning means to you?
[00:09:48] Reuben Tan: Okay. I believe it's just sharing what you have done in your career. What to learn in that program? What kind of skills did you pick up? Maybe in a more data sense, it's let's say companies too very young and like their data journey. So then let's say they [00:10:00] send a couple of guys to go and try to do a simple classification problem and then these guys, maybe they're from the business background. So they study to explore and they come out with some kind of simple regression and then what they can do is they can actually just go and share with the rest of the colleagues like, Hey, this is what we did. This is how we did it. These are resources. Then they can say Hey, does anybody else thinks that this of this framework that we have done this whole system that we have built in the company itself, can it apply to other things? And then you build values in two ways. One people learn things. Number two, that's potential for other products to benefit and more work to be done and better to be added by this stuff.
[00:10:38] Andrew Liew: I see. So basically you get people to share their past projects, like what works, and what doesn't work. And so that the good learning practices can be transmitted across different people. I thought about that, about learning and technology you have typically two schools of thought. One is we centralize all our technology stack and we, I don't call it force, but we make it compliance for people to learn these stacks. And the argument for that there is economic of scale, and better control for the CTO . But then in the world, like you say that we are living today, a lot of things are changing, a lot of technology is changing and it is actually much slower to move it because imagine that you have a few thousand or few hundred people using a specific stack and suddenly you wanna move them. It needs a lot of preparation, and orchestration, and maybe the stack is too old. So therefore no new people are willing to come. They're like, wow, people are now using like Python or Julia for AI. Now you're still using a C plus or some ancient language. And therefore HR will say, Hey it's very hard to recruit people for this old language, then the other extreme school of thought: where the more liberal CTO or CIO says, Hey what is we call it the, bring your own device or bring your own tools, meaning to say that, okay, you have been the cutting edge in learning, let's say NLP and Python. We don't have Python, but so long Python doesn't infringe any risk to my company. Just bring Python in. We will pay for it. $500. $2,000 is. Immediately in stock without writing a 100-page document on why you need to introduce Python to the system, a very decentralized way of, for any software, as long as within the compliance limit. So these 2 schools of thought what is your preference and why?
[00:12:23] Reuben Tan: I think most people would prefer the second way to be able to just do in whatever they want because one there's gonna be a familiarity if I'm certainly asked to I have done R before, but honestly I haven't touched it in a very long time because almost all of the data science community machine community has been pivoting to Python and I think there some migration towards Julia, but it's still in the early days. I was definitely willing to learn if it's required, but that said being able to deploy something fast is also of value. And I feel that being inflexible is just gonna cost you in the long run and like you have said in your example also, like if you're still running something that's very [00:13:00] old Fortran. None of who's gonna code in fortran now. Nowadays you need to move on. Get into python like everyone else, moving to Python through Java script, really. And also there's gonna be the right tool for the right job and you don't want to reinvent the wheel. So now you're the host of a website. Sure. I think you can do it in C. With so many Java script frameworks available. I'm not just picking one of them and let the developer choose. I believe should have enough trust and confidence in your lead engineer to be able to go and evaluate all the styles of things by themselves as well.
[00:13:28] Andrew Liew: So you're also a believer, like decentralized bring your own tools kind of stuff. When, like you say, if you're an expert in Python and it's a cutting edge and eventually bringing in it's approved and this also over time will be centralized because you're bringing the best practices over time to the company. Now coming back to learning. Tell us more about what is your most favorite book that you read for AI or digital transformation or anything, what is your favorite book that you go to or you read recently and why?
[00:13:58] Reuben Tan: I see, I actually don't really read books on AI. I look more, I look at my tutorials in that sense, so I can share a view of my favorite book instead. Yes. Basically, go ahead. Okay. Sure. Yeah. It's literally Freakonomics
Reuben Tan: Sounds about right. Yeah. I just find it very interesting because it's just common sense, but the state so obvious. And it is done in this economic framework in mind. And the key message is just people respond to incentives and not just people. Actually, one of the examples from the book right is about this dolphin, I think they were treating the dolphin to pick up rubbish. So when the dolphin picks are rubbish we would just give the dolphin food. So one day this dolphin kept the rubbish underneath for rock. Then every time he runs food just take a tiny bit of the rubbish, it brings it to the keeper and gets food this way. So I just finished out things very interesting on how people think.
Andrew Liew Weida: I like that book too. I think incentives are the concept idea that [00:17:00] people respond to reward and there are many ways to structure rewards and there are many ways to understand how people respond to reward and by understanding that actually, it helps us to better even figure out how to deploy an AI solution. Figure out what is in it for everybody in the stakeholders to get the data that you need, figure out the problem set, like who are the users, how to figure out the incentive to get them to use, to get the data or how to get them, to use it in a way that it gets output.
Reuben Tan: Yeah I believe that leadership is actually being able to align everybody's fine and goals. So you need to see what inside people have you, okay, this guys wants this to be done. This guy needs this, but this guy cannot have this. So if that's the case, then how do we go and reconcile this of things you need to be able to bring forth people together to move us towards a common vision.
Andrew Liew Weida: Yeah, definitely. Now the next interesting question is what is your favorite application or favorite software that you use most of the time are you recently used and why?
Reuben Tan: I think for me, my favorite software would be Python. I think it's just indispensable to any data scientist. I think it's very fast to write then, and it's very simple. It's not overly cluttered and it's very fast to deploy. I like the language as well. I think I like the guido philosophy of making it a priority. It's really very nice to read and I find reading Python code very easy to understand what people are thinking, and what they're trying to do in the code if it is done properly..
Andrew Liew Weida: You like Python, but what about the ID? Like in terms of the environment
Reuben Tan: okay. I personally, I use I use spider because I had used R before previously, and even before R I used Mathlab. So there's always this tool at the top right hand corner that will actually show me all my current variables. And if I'm doing the data frame mechanism, double data frame, open it up and it's a bit like an Excel thing. Personally do not like Jupyter because I find it very troublesome when I try to travel. So I need to create a new cell. I just wanna print, I just wanna run a single line of I don't to print. I don't run the entire block and Juypter does not allow me this flexibility to do it fast. Whereas spider does just option to run just a single line. I know quite a few before they're actually running VS code and pycharm. I think pycharm is good. I've actually seen [00:19:00] people use it before, but I just I just didn't want to pick it up because I felt that I still prefer spider. Has it something that I've been using for very long already?
Andrew Liew Weida: So you like spider because you think it's easy to use and you're using it, you build a lot of mastery and proficiency. Juypter. Yeah. Even though it's open source, even though it's free, but Like the user interface creates a lot of effort, like you mentioned before. So for anybody like the fresh graduates or anybody who just wanna mid career switch into AI space or digital space, what are the top three career tips that you would think that will be useful for them now that you're into this space?
Reuben Tan: As someone who didn't come from a CS background, I think a lot of people will probably be facing the same situation that I am. Those they already in computer science, they would just be, I do computer science. I can just go and get like role in computer science. Those that are not, and those that are considering joining, I think the first piece of advice would be that tech can be very hard and it requires a lot of patience. Cause they're not giving and you need to be daring. You cannot be afraid to fail. You just need to keep [00:20:00] trying, especially in your own, the development environment, IF you hit a roadblock, don't be afraid to ask for advice from your seniors, your peers, and so on. And I feel like you should join the industry due to the potential that you can bring to others, not because of the current high salary. Otherwise you'll probably experience burnout. My second piece of advice is that after a certain point of competence the most important skill is communication. How you can communicate to other developers, architect, business users, and even across cultures, because nowadays many businesses, they spend multiple countries multiple times and so on.
Reuben Tan: So being able to communicate purely and effective fee, what you want and what you need is a very important skill, as well as being, not just communication of what you want, but what others are trying to tell you. And I believe one of the skills that I use is actually to diagraming up because it just focuses people, it's just a very good way for everyone to get sync. Everyone has a reference. Everyone starts at the same point, just get diagram. And it's a lot easier to get people, to try to understand what they're trying to see. Alright. The 3rd thing is that we should just keep in mind that at the end of the day, tech is just a tool and we are ultimately problem solvers. There's a huge potential for many things to be done out there. And tech space is not just about coders. There's a lot of different, other different roles, like project managers, business analyst, architects, and so on. If you have an interest in tech sector, but you find yourself not very good at coding, you can consider exploring these roles as well. And. I believe if you will, if you are really interested, you can be a PM and you don't just manage the project. You try to actively engage your developers. You try to see what framework are they using? How are they writing code? How are they structuring for? And then from then on you try to learn as well. I believe this will help you make a role better in the future you will be able to throw up some real advice for your developers. Even if it's not constructive, they at least be thinking like, oh, okay this guy knows what he's talking about. And sometimes it is constructive. Hey, why didn't I think of that? This actually makes sense. I can actually explore alternative. A bonus advice. So not just tech it's for just business and life. If you have to add value, you find a problem and you provide a solution because that's where value is created.
Andrew Liew Weida: The first one was like to enable competency you advise people to learn coding and. Have a fearless approach to, don't be afraid to make mistakes. Don't be afraid to make error, just keep going. And eventually you get mastery or at least the attitude of mastery over time because a growth mindset is the same. The second one was don't ignore communication. Like being able to communicate with people gets you easily understand. How things are being done gets you easily set expectations. The third one is the tech is just a tools and we are problem solvers. If you're able to solve problems and whatever language or whatever tools, then that way it enables anybody to go to take a career in AI would be easier. And the fourth one is if you wanna add value. Find a problem and add the solution. Cool. So these are the four ones.
Andrew Liew Weida: What is that one ask that you want to get from the audience who are listening to this podcast show? What is it that you want from this audience?
Reuben Tan: Oh yeah, sure. If anyone is interested to know more about what I've done. Connect with me. They can add me on LinkedIn. Yeah. Cool.
Andrew Liew: I'll share it with the audience . So thank you so much. Ruben, it was great having you in the show.
Andrew Liew: Hi everyone, thanks for tuning into this episode. We have come to the end of part 4 with Reuben
Andrew Liew: and this is the end of the podcast series with Reuben.
Andrew Liew Weida: If this is the first time you are tuning in. Remember to subscribe to this show. If you have subscribed to this show and love this. Please share it with your friends, family, and acquaintances. See you later and see you soon.
Source: Freakoonomics: https://www.youtube.com/watch?v=LrceH3952Hg&t=37s