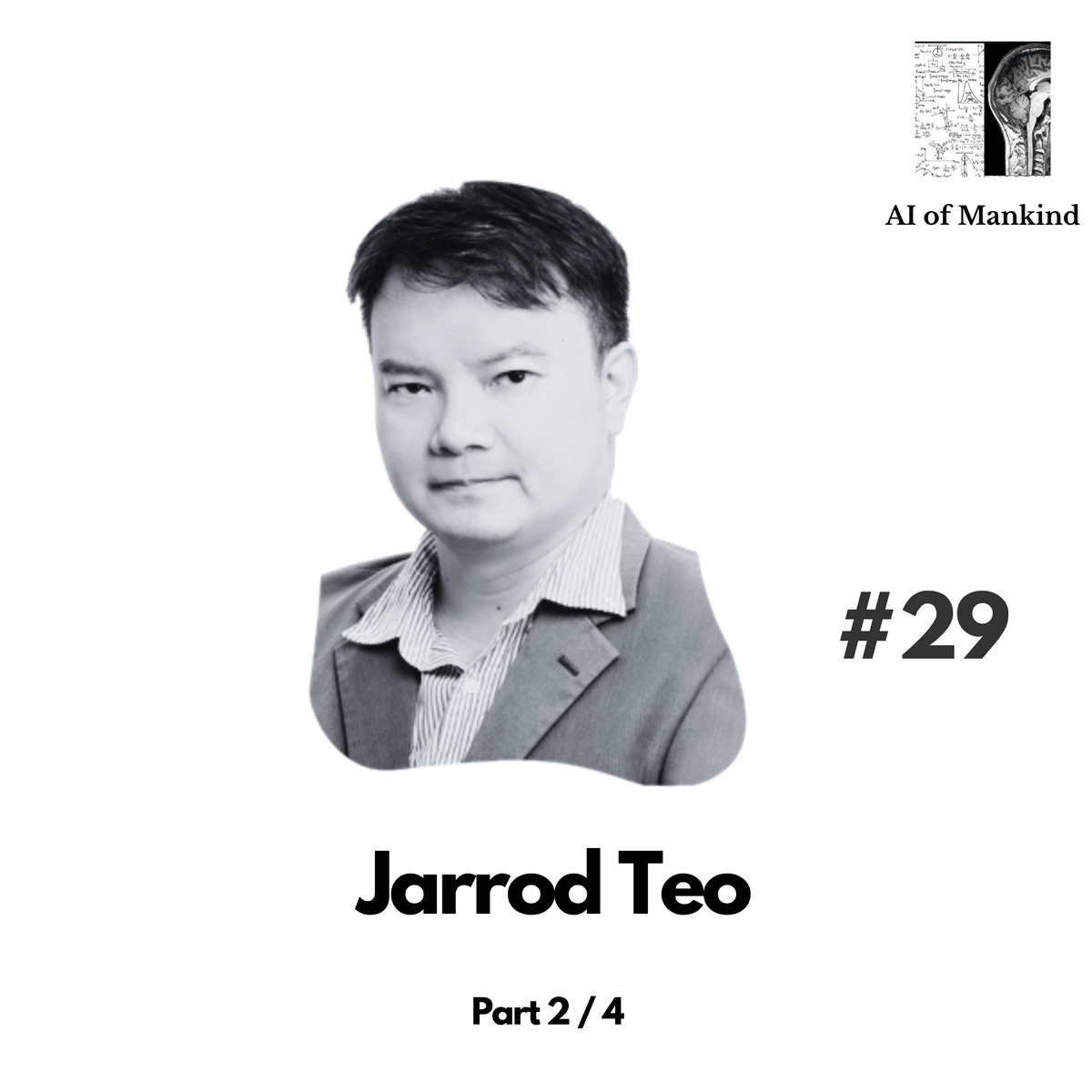
Trailer
Podcast with Jarrod Teo Part 2
Summary:
Jarrod continues his conversation, sharing digital and data transformation case studies, including one on helping the government with volunteer acquisition for non-profit organizations and another on a successful digital marketing campaign in a country of unrest. He also discusses a project that raises ethical questions about privacy.
[00:00:00] Andrew Liew Weida: Hi, everyone. Welcome to the AI of mankind show where I share anything interesting about mankind. I'm your host for this season. My name is Andrew Liew. I work across four Continents and 12 international cities. Also, I work in tech startups across a range of roles from selling products, making customer happy, figuring out fundraising, making finance tick, building teams and developing sticky product. Apart from building startups. I've also worked in fortune 500 companies as a chief data scientist or technologist or people leader. You can call me Jack of all trades or master of learning. I hope to make this podcast show [00:01:00] a great learning experience for us In each season, there is a series of interesting things where invite guests to share their views about their life and interests.
[00:01:09] Andrew Liew Weida: Now let the show begin.
[00:01:26] Andrew Liew (2): In the previous episode, Jarrod talk about his backstory. Along the way, he mentioned about his journey to picking up statistics, taking up a job with IBM SPSS as a trainer to eventually becoming the Chief Data Scientist for a Direct Sourcing company. This episode continue the part 2 conversation with Jarrod and Jarrod shares his digital and data transformation case on helping the government with volunteers’ acquisition strategy for non-profit organizations. He also shares a project that makes money at the expense of others’ privacy. At the same time, he shared a successful data transformation story of how a digital marketing campaign is done in a [00:02:00] country of unrest. Let's continue.
[00:02:02] Jarrod: First question. So then, another thing is that I did actually manage to train to to, present, not to prime Minister Lee, but present to a lead family member. So I did actually present a statistical model to Lee family person. And then that one was actually helping the company to actually find out why volunteers don't want to actually volunteer at all. How do we attract more volunteers? Interesting. Yeah. Yeah. So
[00:02:33] Andrew Liew Weida: I and, tell me what was the thought process then?
[00:02:37] Andrew Liew Weida: Like, what kind of schools are taught about people volunteering or not volunteering? What are schools are part there? What
[00:02:42] Jarrod: reason? Yeah so, basically what happened was that the company don't know the face of the volunteer. It's is always happening like that. So they don't know the face of the, they don't know who they are and all those things. So, then I have to actually profile them and understand who volunteer and who don't [00:03:00] volunteer. If they
[00:03:01] Andrew Liew Weida: don't know who they are, how do you collect the data?
[00:03:02] Jarrod: They have the, data, without data, there's no science. Exactly. So they understand who the volunteers are. They just don't know who they are in terms of the profile. They, have some characteristics now. Yeah. So then I actually go and profile what makes a volunteer, what makes a loan volunteer. So once we know what makes a volunteer, what's make one, makes a non volunteer, I found out with some division three and I was actually going into even to find out what kind of activities the volunteers are actually taking up. What kind of activities? Activities, the non volunteer use to take up. . So these are non volunteers now, but they used to be volunteers, right? So they used to actually take up some activities before. Yes. And then I found out that these non volunteers tends to be those that are workers, like maybe say 40 to 50 or 30 to 40 range, that kind. And then they take up things that is simple to do. That is, we say on weekend a few hours, those [00:04:00] that is younger ones that is actually 18 to 20 plus and 18 to 25 they, are actually taking up more time consuming those that need more time volunteering work like maybe say the entire morning or the entire weekend and accounting not one or two hours. So then I point this out to that volunteering company that volunteer non-profit organization. I was telling them yes, I said that. So this is actually something that you need to take for the volunteers and non volunteer. Then you, since you know who they are, you know what they do. You want to know where to hit them. So then I ask them to brainstorm as well. That is, so where do you think you can actually find someone that is young and then do all these things? Someone that is actually to be white collar where to find them for simple task. on Saturday where they can actually just one hour maybe say take some kids out for a walk from those lower income family. Why, not? So then you, must know who they are first, then [00:05:00] you know what they do, then you know where to find them, then that might actually increase the chance of hitting a volunteer.
[00:05:06] Andrew Liew Weida: Talking about volunteering, because I remember I was having this chat with a few previous podcast episode. One of the things that, one of the data scientists was saying that because he does international benchmarking work and he was saying that actually countries that has a, better welfare state that let's say promote more allowances, I'll call you universal benefit income or unemployment benefits. There is a higher degree of people or population to do volunteering because they don't have to do a very hard trade off between work and volunteer. That's right. And, so is there some kind of true on that based on your current research for that project? Is there something that you guys discussed about that before?
[00:05:50] Jarrod: No, because we don't have a direct data to actually point to that. Information that one, if you actually want to come to the conclusion, you really need to actually have a [00:06:00] very, solid based database to actually come up and say, oh, this is how the entire country is actually behaving or things like that. Because that is actually a survey form collected data. And yes, that database is her copy . Wow. Yes. Not all database. I actually soft copy. So you haven't, before how, do you deal with
[00:06:20] Andrew Liew Weida: hot copy data at that time? Oh, wait how many years was that?
[00:06:23] Jarrod: That was 10 years, two years? How many years back data That was around, I can't remember. Maybe say seven years ago. Seven years ago. Oh, 10, 10 years ago-ish. Is half copy data. Yes, of course. Then we have data clock to actually enter the data into ah, okay. Spreadsheet. Then we start to actually do the analysis and things of that. Yes, I seen database, in. Paper form, right? paper form. It's actually horrifying .
[00:06:53] Andrew Liew Weida: I, had the same experience. That was about, I think 2003, almost 19 years ago. Yeah. When I was [00:07:00] a junior data analyst for a big consulting brand. And I was like, oh man, we are gonna work for this, I think some chocolate company. . And then we went to the to the office. We was so excited. Ah, just bring my laptop a little bit easy. Then the guy, the HR bring one step, oh, this is all the payroll data. I was like, wait, this is in paper for Yes. You gotta first enter the data, the benchmark. And I was like, oh my God, this part, something. Literally just enter take picture and transport the data.
[00:07:38] Jarrod: Funny things actually. That do happen in, our line of work as data scientists. I can actually jump a bit on to talk about some of the work that I had, the unique ones, which is actually you URL browsing protection model. So the one that you browsing prediction model is the last phase of the entire telco work. I hate that model.[00:08:00] . I regret building the model because you are on a browsing protection model. It runs for six years, actually earning money 24 7. I should be happy, right? But no, because it is actually looking at everyone's privacy. Even https they can actually dig the information and then go and find out, so why you actually serve account website you serve. And then from there we'll actually find out the URL and app yet to use and then find out what TV channels to sell to you and is across all the
[00:08:30] Andrew Liew Weida: Browsing tools like, like Google, Chrome,
[00:08:33] Jarrod: like mobile or wifi. They can catch you. Yes. So I, don't like the model I was asking my client. I say, is it actually still functioning? He say, yeah, it's still functioning, but then it's we are trading off our privacy for money. Yes And that is the last phase. The first few phase was actually trying to. Whether the person is active user, whether the person actually increase in usage what can we sell him next? Can we [00:09:00] actually sell him more services, which is mobile to wifi or wifi to tv? If so, then what kind of TV channels can we sell to him? Can we refine the TV channels based on the URL app that the person that you saw? Yeah. So it goes all the way there. That's the last phase. And some something, interesting actually happened. So this is actually, we were doing the cleanup of the url, and of course we have machine to actually help on that. Let's say that you are actually serving YouTube. I serve YouTube and then we, should actually be, classifying YouTube as entertainment. But because you serve a lot of golf, I serve a lot of games, video games. So I have a lot of banners actually appearing in in that YouTube itself. And then you have a lot of sports banner appearing in the YouTube. When we actually call information, the entire paragraph of words actually is yours is golf, mine is Gaines, but we want everything to be classified as entertainment. So then, I was telling my [00:10:00] friend, who is actually a tech scientist, that you should actually look at how we can identify the number of our currents of words. That's up appearing. Entire 300 over words and try to classify it accordingly. I was telling or giving him some sort of like instruction. And then once he actually devi his own method, he gave me the raw data, which actually had that whether is entertainment, whether is sports, whether is law related or business related, then we, start doing our work, which is actually using cays to, to actually classify the, customer. But then that work is a cays within the cays eventually I, don't like the cays within cays because then the error could be square, right? The errors, because the model with the model actually cause a risky error there. But thankfully the model still runs for six years and, then why [00:11:00] we do a cays within cays is cause the time, everyone is so happy about Pokemon Go and social media, especially Pokemon Go. It appears across all the cays social media appear across all the ca all the cays. Then we can't do anything. We just say, okay, let's do it this way. We redesigned the cays, we redesigned the blueprint. We actually had a bigger cluster of cays and then from there we use a cays on the vehicle cluster, some, something like that. Then, once we did that, the model actually comes up with interest. Combinations of URL app that a person used. But something happened in the project that was actually quite co ki interesting. Now there are actually some strays URL that is actually not classified properly. And that we have things like location of the person the airport location that the person serve. So I have to actually go and classify manually how to actually this is actually some strains url. So I have to actually go and classify location models. Then I have to [00:12:00] classify medical information. So one day, imagine one day, one day we are actually airport specialists. Second day we are actually doctors sitting in the room trying to understand what URL is actually talking about. It's actually about knee pain. Is it have a heart pain or ?
[00:12:18] Andrew Liew Weida: So talking about that you are thing and, the IP address, if the end user or the user V p n that constantly randomized a v IP address or Yeah. Constantly change the browser, the model will break. Or you, mean you guys actually account for all these factors
[00:12:34] Jarrod: as well? We, can't control the v p N part, but then so long as the information is there, we will use the information to actually do the The classification in orders. Okay. So then, something really interesting happened that is we were actually asked to actually classify porn website, .
[00:12:52] Andrew Liew Weida: What was the classification by?
[00:12:54] Jarrod: So, he say that, porn website is something which the client [00:13:00] wants to take out. So Jared, these are actually some of the stray url, which we cannot classify, whether it is porn or not porn. So can you click on the, link and then just go inside and see whether it is porn, if its you put porn, if it's not porn, manual label. Really? Yeah, I would, it's some stray. It's just some stray website. So it's just imagine just one spreadsheet of links. But I was saying, but I was telling my research director, look it's office. How do you want me to actually just serve this website in office? Open up and then, yeah, it's office. So, she say, just book a room, go inside a room and then just enjoy yourself. But , I was like no, What if someone walk in the room and then yes, someone actually go in the room and they're ladies, wow. So, then we, work from home and to make sure the classification is done properly. , it was very funny, but [00:14:00] it was actually done. And the client first reaction when he actually see all upon your album being captured properly, he say, let's. Upon services we earn, like math, , you have all the things there already, , but of course they actually can say everything out so that he is joking. Aye, he's just joking. He's just as a telco company. They want to ban all this website so that people will not actually go into this website and then, yes. That's why some national
[00:14:29] Andrew Liew Weida: Not American company now is probably some country that's very conservative, right? Yeah. Because imagine in the US in Japan, you depends on this kind of industry to prosper your
[00:14:39] Jarrod: telco business. So then, what happened was that we, finished the work it was very funny. So we, eventually finished, the work and then the year round app browsing protection model we were testing it as well. And then we are very worried about one thing because this is actually mobile versus wifi. Now [00:15:00] mobile is actually very personalized. So it is something which you, we are not actually deviate so much. Yes in terms of the profile. But wifi is something that we are worried about because it, can happen in such a way that people come to a house and then you suddenly needs to actually that profile weight suddenly change, right? Because the person come to a house and serve using a. . Great. So we, go by the law of large number, thankfully because the person, the owner of the wifi is staying there for very long, but a few strains of wifi usage won't actually change the characteristic that much, right? So we are, we're somewhat right about that. Until, we tested and we realized that Malay 80 years old was used to serve video, right? Video call, and then suddenly she start to serve parents and parenting and e-commerce. Then we were very surprised. Then we thought the model is wrong. Then my research [00:16:00] director said, don't panic. We actually see what happened in the third scoring. So on the third scoring itself we realized that it become video call again. Then, I was telling my client, I don't have solid data to prove this to you, but looking at the calendar, if you actually notice the behavior of the wifi why this Malay 80 years old is doing like that is ah, solo, ah, and then her kids should actually have came back to her home. And then they have younger kids of their own and they are fasting and so they're serving parenting website and then they're using e-commerce to buy cookies. , then it actually goes back after Aria. Go back to go back to video call. Then my client was actually quite impressed and say, wow, Trevor, your machine learning model actually takes care of festive season
[00:16:52] Andrew Liew Weida: about this. Yes. How do you pick out this, I call it out of the context or the nuances to be able to read [00:17:00] this thing and train the model? This is why I call business a human. Like how do you develop that? Or do you think that it was true your career or you, learning
[00:17:08] Jarrod: somewhere? It's actually true. My career somewhere down the road in ibm m SB says consistently talk with the business people. Then it, trains me to be a front end data scientist. So, which is back to what I was saying that then somewhere down the road you have to actually decide whether you want to actually become a front end data scientist. And your challenge, of course is this, that is, can you set up the department from scratch? That is ground zero, no data, no software, no hardware then you have to actually test your hardware, test your software, which one you want to use what is actually the p and l profit and loss that you're expecting. Of course, the cost will conference because you're the set of the department. Then what kind of people you wanna hire? What is the procedures of data process that you are looking at, extraction, cleaning you to set up the cleaning process and all those [00:18:00] things as well. Then all this, after you set up, then you will want to prove your models and all think or work analysis they will use. This is actually another what I can say, phase of a data scientist. This is after a senior data scientist or maybe a data science manager onwards whereby he lo he don't look at just modeling and coding anymore. He also will look at the entire package, which is actually the p and l of the data science department. Profit loss. Yes. Then after you set up the department, then hopefully you will not face this, but if you face this, then congratulations. This is actually another phase for you, which is to deploy a model in a very southern economical event. . So I faced that four times really. So I'm sure some of the listen economic event means. Yeah. I'm sure some of the, I'm sure some of the listeners faced it [00:19:00] as well. So the first one is actually thai protests, country unrest. I have deployed many models, but then deploying a model that earn money during a country unrest is something that is first time for me oh.
[00:19:12] Andrew Liew Weida: Sh share with us the, general idea of what actually happened or why, was this so interesting or what was so stressful. Okay. Yep. Okay. Yeah, sorry. So you, we were talking about you're trying to give advice to a data scientist or data analyst or somebody who has set up a team to become a manager, and you are talking about one of the trigger events, Thai protest, or country unrest.
[00:19:34] Andrew Liew Weida: So I tell us a bit about that story of that. how did it happen or what happened and what, was going on? What do you have to do and how do you solve the problem?
[00:19:44] Jarrod: So Thai bureau was actually a unique situation. Something which we didn't expect.
[00:19:49] Jarrod: I was actually telling the vice more. I said we actually just push the big sales event to a later date because we, aren't even sure that our [00:20:00] delivery van is able to actually send the products to the customer. . Yeah. Because this Thai protest, everyone is blocking the, street they take over the airport and whatever.
[00:20:07] Jarrod: So, then my, I see more saying, Jerry, it's time to test your model . Yeah, I know. I had that same expression as you the, face was like the mouth open wide. I know, right? Oh my God. Yeah. Are you sure? So then we, we, did it anyway and, we did a few tests before the actual launch. Thankfully we did it before the actual launch. And we, yes I always actually test my model before we launch it. So we, had predictions said that these people are by fashion product, and then we send first group, A, we send fashion. Could be we send garbage. So then we, just wanna make sure what is garbage in this context? It's random, product, . So we call it garbage. So we say random product. We don't send any fashion we send because all these are predictive fashion. [00:21:00] So we go extremely, because all these people should buy. And we are saying that if we send them non-fashion product, they shouldn't buy anything, so, if the non-fashion group the, non-fashion information group, beer, they still buy. Then the model is a failure, so the open rate is the same, the click through rate is the same, but the conversion for the one that is actually been sent fashion is twice the amount of those that are sent garbage.
[00:21:29] Andrew Liew Weida: Yes. It's very interesting because normally the, like the garbage here is like the control factor, right? Yes. And then you have this like changing factor or boosting factor. So in this case you almost flip it
[00:21:39] Jarrod: around, right? My usual word is a group is we is fashion buyer. B group is really, we send non fashion buyers. But my director at the time here has a very funny, she has a very funny thinking. She say, we just choose all fashion people and we will send group a fashion group, pe, non-fashion. , [00:22:00] she wants to prove a point that my model will fail because these are actually buyers anyway. You send anything, they'll buy, right? But then that campaign actually prove her wrong because when I say that she buy the person buy fashion product, they buy fashion product, it's twice the amount, then those, that's actually sending non-fashion product. And
[00:22:22] Andrew Liew Weida: what was the response from the stakeholders when they saw the result of their first trial?
[00:22:26] Jarrod: Let's actually get Jer send more and then I send more. And then the Thai themselves, the company, they send 8.5 million emails back, 40 K tibar. Ah during the protest, period, I send 9,000 email. They send 8.5 million. They earn 40 K Tibar in a manner I send 9,000. I unpack 100 K in a week, in two weeks or in a week, two weeks, .
[00:22:54] Andrew Liew Weida: And before that, what was their business as usual? Experiment. Let me,
[00:22:59] Jarrod: they [00:23:00] send, they just messy send emails every day three times a day. every week, consistently. Every day we send three times a day. So that's why you chalk up to 8.5 million and then it chalk up to 40 million. It's just actually just 40. So not 40 million, 40 k high, but only I send 9,000. I earn back 100 K in two weeks. So they take one month. I take two weeks. So, they say general continue then, so you know, the thousand, the 9,001 is actually the type test start of type test B right now. And then we actually send now 9,000, we earn 100 K itself. It's whoa. Okay. So my vice CMO say, is it that Thai protest means we'll have bigger sales? ?
[00:23:46] Andrew Liew Weida: No. Are you selling shirts? He was like, this brand type protest. ,
[00:23:52] Jarrod: no, we're selling different thing fashion, food, whatever. So then, we send to an entire month I was sending [00:24:00] around I think 300 K emails and then we, earned back 400 K type in the entire month, which is 400 K, which is actually really, literally a lot of times more than their 40 k type. And then they sent 8.5 million. No, I only sent like what, 300 to 400 K emails and then I earned back my 400 k typer .
[00:24:20] Andrew Liew Weida: Was it also because the email that you got was like what we call m pq, market qualified leads. That's for them. They just buy a bunch and then pre and
[00:24:28] Jarrod: spray it. I don't, yes, that's actually the case as well. But then I was also sharing with them that what you are sending even you're sending to your qualified customers. Yes. They are actually sending information that your qualified customer don't like. So I was sending them information that they'd like to see and then because of that, then the ties there is, there is the ties the magnetic pool of buying is there. And then I was actually sharing as well that the reason why is it like that is because also [00:25:00] that then they will not actually trade to junk box. They response with a purchase as well. . So, then this actually shows that really customers really want to actually see a customized view of what they want to buy. If you go to certain supermarket. You actually look at the receipt print behind detergent, mop oh, yes, I see ads. . Yeah, ads. But then I'm not enticed by detergent, mop web. I see continuously over the years detergent mop. It's the same thing and, dishwasher. It, doesn't entice me at all. I'm, looking for snacks or something nice. But then it's not customized to me. Why should I actually be ties with something that I don't like to do? Detergent, mob, dishwasher. I do housework. I don't like to see you have
[00:25:49] Andrew Liew Weida: There's , two schools have taught in marketing, right? Yeah. One is do branding just keep just true at the user? Like, in this case behind the s this, like you said, washing, machine washing [00:26:00] powder, whereas you feel this is the second score to just be target, just shoot where the bulls are is, right?
[00:26:06] Andrew Liew Weida: Yeah. And what do you see like in, in, the world of marketing or, any clients that they are moving towards the second part of the booza because the branding, the impression just high frequency will work. I don't know what, are your thoughts of this?
[00:26:20] Jarrod: So you see this is actually the, very interesting discussion I had with the senior stakeholders inside the company. Certainly they say that, look, Jared, I know my competitors are targeting their clients very well. They're also targeting my client. And not only that, my clients are buying from them before they actually know what they want to buy. And then that actually means that they have no money for my product. So they were saying, Jerry, is there a way for us to actually know what the customer might buy be before they buy? Or can we actually target them a certain product? And they were also [00:27:00] consistently conscious about this. That is Jerry we spend a lot of money on discount for marketing, right?
[00:27:06] Jarrod: Yes. We just actually spray and pray by sending discount to everyone and think that they'll buy, and I was actually telling them, say that the strategy is, needs to be tweet a bit. Those that are actually your premium customers, they'll buy anyway, discount or not, right? Why don't you actually keep get this discount and then save it and use it on those customers that are actually almost leaving the company. Or use it on customers that are actually above already left the company and get them to come back. So then you see, then your, discount is actually saved and utilized in a more efficient manner rather than actually just spread and pray to everyone. So in order to do that, you need to actually know who are you targeting, what are they buying and, things like that.
[00:27:59] Jarrod: [00:28:00] Then the, they are actually very attuned to this one because they're saying that up to now they are strategy is that the person who spend more means they are likely to buy more, right? But I've given them a score of thought that is yes, they can spend $1,000, but then what happened if you spend $1,000 today versus someone who spent $1,000 a year ago, which one will you treasure as a customer?
[00:28:29] Jarrod: The answer is obvious. I'll tr I'll treasure those that can give money now better than one year ago. So then you see then the, majority thinking that, oh a high spender means that he's a treasure customer is no longer there, right? You must actually see whether he can give you money fast enough so that it can become it will, can solve some cash flow issue in the company, and also can actually get them away from your competitors as.
[00:28:57] Jarrod: And, you are in this digitalization [00:29:00] war that it cons that is consist consistently going on is a war that is going on online Now, that is, we will never know whether the customer are being targeted by your competitor competitors or so. Yes. That's, and then they could be using it across the street in, their retail store and say, calling up your customer and say that we'd like to buy something from us
[00:29:23] Jarrod: So this is the situation and, this is the country and rest story that I had that I bring and to actually talk about more about the advancement of the data scientist after you actually had done the country on re the next one you should look at is whether you can do a product innovation.
[00:29:42] Jarrod: Yeah. This is very difficult because you, if you, because normally as a data scientist, we are trained from bottom up. We are looking at how do we extract data, how we transform data, the data in orders. But suddenly as a person who is actually doing production innovation, [00:30:00] we have to change our score of top to become from top down, which is from what the market needs to what data we have.
[00:30:08] Jarrod: Yes. What methods we, should carry and what, data we have. So, then once you've reached this stage of product innovation, You're somewhat ready to actually present to investors, which is to dive inside a shark tank . So if you can dive inside a shark tank and, still remain alive and and still can smile, then yeah, you are somewhat there if you can I, went to present to layman merchants vegetable sellers who are trying to sell pickles online, , and yeah, vegetable sellers.
[00:30:48] Jarrod: Even investors who actually just want to understand what data science innovation are you going to sell. So if you actually start to talk, them about, oh, he's adjusted R Square. Oh, you're using [00:31:00] Python? Oh, you're using XG Boost. That's it. They just say, I'm sorry. It's very interesting, but then I don't want to invest
[00:31:09] Andrew Liew Weida: that story.
[00:31:10] Andrew Liew (2): Hi guys. Thanks for listening to this podcast. In part 3 episode with Jarrod, Jarrod and Andrew exchanged views on the difference between a data analyst and a data scientist, the difference between a technical data scientist and a client facing data scientist. Andrew shares a trend that companies are seeking data strategists to build data roadmap and data blueprint for companies and Jarrod mentioned the need that companies do not just stop at that stage but follow up closely to implement the roadmap and blueprint to avoid a translation gap. Both agreed that companies need to be patient with implementing data transformation as Jarrod share a funny story about Twitter wanting a machine learning model in the next second. If this is the first time you are tuning in. Remember to subscribe to this show. If you have subscribed to this show and love this. Please share it with your friends, family, and [00:32:00] acquaintances. See you later and see you soon.