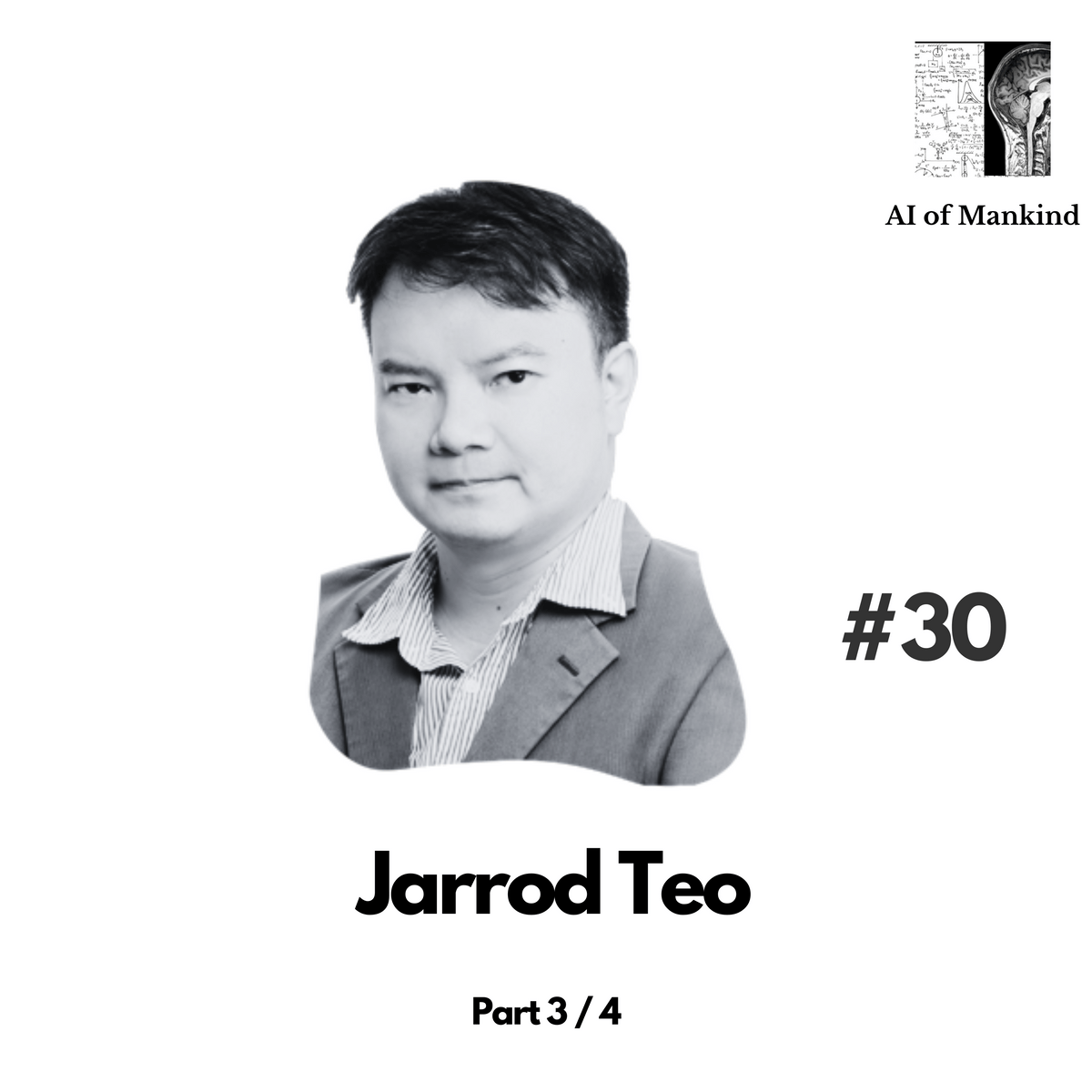
Trailer
Podcast with Jarrod Teo Part 3
Summary:
Jarrod and Andrew talk about how a data analyst is different from a data scientist and how a technical data scientist is different from a client-facing data scientist. Andrew shares a trend of companies seeking data strategists to build data roadmaps, and Jarrod emphasizes the need for companies to closely follow up on implementation to avoid a translation gap. Both agree on the importance of patience in implementing data transformation. Jarrod shared a humorous story about Twitter wanting a machine-learning model too quickly.
[00:00:00] Andrew Liew Weida: Hi, everyone. Welcome to the AI of mankind show where I share anything interesting about mankind. I'm your host for this season. My name is Andrew Liew. I work across four Continents and 12 international cities. Also, I work in tech startups across a range of roles from selling products, making customer happy, figuring out fundraising, making finance tick, building teams and developing sticky product. Apart from building startups. I've also worked in fortune 500 companies as a chief data scientist or technologist or people leader. You can call me Jack of all trades or master of learning. I hope to make this podcast show [00:01:00] a great learning experience for us In each season, there is a series of interesting things where invite guests to share their views about their life and interests.
[00:01:09] Andrew Liew Weida: Now let the show begin.
[00:01:26] Andrew Liew Weida: In the previous episode, Jarrod shares his digital and data transformation case on helping the government with volunteers’ acquisition strategy for non-profit organizations. He also shares a project that makes money at the expense of others’ privacy. At the same time, he shared a successful data transformation story of how a digital marketing campaign is done in a country of unrest. This episode continue the part 3 conversation with Jarrod and Jarrod and Andrew exchanged views on the difference between a data analyst and a data scientist, the difference between a technical data scientist and a client facing data scientist. Andrew shares a trend that companies are [00:02:00] seeking data strategists to build data roadmap and data blueprint for companies and Jarrod mentioned the need that companies do not just stop at that stage but follow up closely to implement the roadmap and blueprint to avoid a translation gap. Both agreed that companies need to be patient with implementing data transformation as Jarrod share a funny story about Twitter wanting a machine learning model in the next second. Let's continue.
[00:02:21] Jarrod: Even investors who actually just want to understand what data science innovation are you going to sell. So if you actually start to talk, them about, oh, he's adjusted R Square. Oh, you're using Python? Oh, you're using XG Boost. That's it. They just say, I'm sorry. It's very interesting, but then I don't want to invest
[00:02:43] Andrew Liew Weida: that story.
[00:02:43] Andrew Liew Weida: Can you tell us like what, exactly happened? Like, how did it begin? What, was going through your head as
[00:02:49] Jarrod: well? It comes from requests from clients and say, I want something that is this certain product data innovation that actually solve, solved my business problem. And then [00:03:00] we come up with innovation that actually earns that valuation of the company, 13.4 million U s D. And, then that allows the customer to actually find out information from US or China without need needing them to have data themselves . So yeah, it sounds called creepy . So it's possible. Anyway, so that's, actually where the innovation spirits come from. That is then I will consistently start to think about, what does the market what, the market needs? What, can we feed inside the market, which can solve the business issue of a certain industry. Let's say if you are in oil R what is the oil R issue facing? Let's say you're in healthcare. What is the healthcare issue facing? If you are in retail e-commerce, why is the retail e-commerce facing? So if you can actually find a solution that can actually be repeatedly being used [00:04:00] for con from company to company, then, and then you can set it up in a very strategic manner. And the, procedure is like standard standardized in the company. It is almost like going to a product phase rating. And
[00:04:14] Andrew Liew Weida: what was the challenge that. Thing that you were going through at a time when you were trying to build a product, when you was trying to even also source whether the data is available.
[00:04:24] Andrew Liew Weida: Now, it's a very common problem. Yeah.
[00:04:26] Jarrod: It's common about companies. Yeah. But then what, I did was, so I was, consistently start to actually think about, this is actually a, business problem then is it actually a data which the business is likely to have? So when you actually work in that industry for quite some time, you will know, for example, an a credit card company definitely will have credit score information.
[00:04:48] Jarrod: Yes. Definitely will have customer information. You can't run away. The bank will have customer information. Yes, The bank will have transaction on the cut itself. Yes. Then can you actually create a product out it? Yeah. And then [00:05:00] what is the difference between a project and a product? Is that a project actually?
[00:05:04] Jarrod: You build on a spot for them. You hands off and then tell them what's the model and then that's that, right? Yes. But a product is something which you can actually ask them to buy maybe say on a subscription basis. Yes. And then it's not a hands off, you don't need to tell them what's in the model and then they'll actually just be a subscriber to the, that product that you had and then it's yours forever.
[00:05:29] Jarrod: And then it's not just a one-time more project anymore.
[00:05:32] Andrew Liew Weida: Ah. So it's almost like I call like embedded analytics or embedded machine learning. It's like this particular piece of work. Whatever product to enable it to have continued with the us. Let's say a project like say in this case for your, for the government, for policymaker, is the insights that take away for them to say, okay, let's do this because it has five years.
[00:05:53] Andrew Liew Weida: Ramification, a daily basis is a different story, right? Yeah.
[00:05:58] Jarrod: So then, to [00:06:00] convince the the investor is a totally different thing altogether because the investor will actually think product as pluck and play. Ah, yes. As data scientists, it can be a challenge for plug and play most of the time, because we need internal data. We need to clean the data. Yes. So sometimes the business people can come to you, ah, our data is perfectly clean. That's solution . When I actually hear that, the data is perfectly clean, that's a red flag.
[00:06:31] Andrew Liew Weida: How, do you come, okay, first of all, how do you sense this kind of red flags? What are the red flags? Because I also still learning sometimes I got, I don't call it corner, but I was like, I learned something. Yeah.
[00:06:42] Jarrod: When they say that the data is perfectly clean, you know that it's a red reflect really. You, don't need to think so much to say, okay, Ken let me have a look at the data. So you see a lot of data company out there, a lot of business of there. They, are very concerned about one thing. Business cash flow. Yes. Yes. What I [00:07:00] think about the data. , they're business people. They, won't, they are not as articulate as us in data. So then they, they, will have this, gap that, which we, data scientists need to breach on the data between them and the data, right? Yes. That's why we are hired as data scientists to breach between the data and them. And this is such a problem. They think that they have a data lake, but then you see multiple 30 data brings together doesn't become a data lake. It becomes a data swamp. ,
[00:07:31] Andrew Liew Weida: yes. I say data, mangroves, right? Yeah. They say we have clean data, but you gotta dec this desination pump, you got clean and processed.
[00:07:41] Jarrod: It be drinkable, right? Yeah. It's, it's initially it can be quite scary, but then once you actually walk through multiple data from yourself, it becomes second to none really. So then you know what to do. When you actually face the data from what questions to ask [00:08:00] what's actually the, sum of the people to approach and how do you approach them to get answers and things like that. Yeah. So I even have a standard procedure on how to clean data and things of that. Really? Yeah. So then it's not easy. The journey to become a data scientist is really literally from data nalytics as. Junior as you can be, just try to actually learn, be patient, learn from scratch, actually go and matter with real data. Do, I suggest joining data competition? Yes, you can join data competition. But then data competition are still competition, right? Yes. You, will see, you'll see maybe say data are pre-prepared for you. Yes. And not only that, that is, you don't see your model being deployed. You don't see your analytics being deployed as well.
[00:08:51] Andrew Liew Weida: No. Not just the deployment part. But like you said, the data is prepared. Even the business case is well written for you. In reality, we don't have the csuite [00:09:00] guide, the street guy, the C street guy, or say for example, I want to increase my revenue. There's,
[00:09:04] Jarrod: so yeah. Wanna money, revenue.
[00:09:06] Andrew Liew Weida: What are you looking at? Are you looking at the, like you said, the product? Are you looking at your business? Are you looking at the people? There's so many ways that data science can
[00:09:13] Jarrod: do, right? Yeah. I want to grow my plants. So , that's actually the CAM is a really a go government chemist that actually had met. So yeah, it is, you are right. That is, even the business objectives are well written for you. So data competition yes, is a good way to practice the things that you learn. But then ultimately still you, used to hands on join a company, be humble, join a company, start as a data analyst and then look at the data proper and then, Clean it properly. And then from there, you try to proceed on to become a journey as a senior data scientist, which actually is understanding how the tech stack flow, right? And then of course, then the journey there will have [00:10:00] to decide for you what kind of data science domain discipline you want actually go into. There's a data science discipline, the five discipline, numeric image, tax, video, vo what kind of industry you wanna focus on. Is it banking? Is it insurance? Is it what kind of industry? Then you well look at whether you want to actually still remain back in or you wanna actually go front end, which you will talk more to business people. You need to understand how to explain things in layman manner. And then you will set up department as well. And then you actually do innovation. Then you also look at presenting to investors.
[00:10:45] Andrew Liew Weida: So tell me about say that your view on, digital transformation or let's say a c-suite client.
[00:10:55] Andrew Liew Weida: He listen to this podcast or he knows you, then you are, Hey I want [00:11:00] to do data science, I wanna do ai. What is your advice for them to think about how to begin this?
[00:11:07] Jarrod: How do we begin this process? Is first understand if you have data to actually begin with first ? I do.
[00:11:16] Andrew Liew Weida: Yes, I do. How do you, ask him and how do you get these people to be aware?
[00:11:22] Jarrod: I, will actually ask him what kind of data that he has and I do actually have two significant cases where their data, it's really very interesting. One don't have data customers. They don't have customer id. Wow. Which means that they only have transaction. So I was actually Is that a,
[00:11:45] Andrew Liew Weida: small company or big company
[00:11:46] Jarrod: without customized it's, a restaurant basically. So it is a certain restaurant in marina Basin that sells hero products though those kind of heroes product. But then it, was closed down. It's quite a [00:12:00] p because that restaurant is actually quite nice. It's actually selling those kind of one of thing of either DC or Mar or one of them. So, I was actually quite enjoying the product and then they have to close down because they don't have custom id. And then because of that they don't, they only know what are the products that is selling well or sell, not selling well, but they already know that. But what w what we want, what they want to do and we hope to provide, is to profile the customer to know what marketing messages we. Send out in a targeted manner to them to actually bring back the customer sustainability or wing back the customer. Yes. So, they can't because they don't have customer id.
[00:12:45] Andrew Liew Weida: Yeah. Yes. So when you say that, you tell them that, Hey, you don't have a customer id, and first of all, do you ask them how come you don't have customer id? And what was the response when you tell them, Hey, you don't have customer id, I can't help you further that.
[00:12:57] Jarrod: I was actually telling them that is there [00:13:00] possibility that you can start collecting your customer? Id first give them some loyalty because you opening for quite some time. You should have able to actually give them some loyalty a card or whatever. Then when you, they come back and then you scan the QR code and all those things, then it's registered inside your transaction which customer, like what kind of products. Then you can directly target the information to them. Then they'll say, okay, we'll try to actually see we do that. Not, then it goes down but we, data scientists are not magician. We, need data for science. You don't have data. We can't do science . That's, the situation. And another, situation is that the company actually come to me and say, Jared, I have a lot of data, 200 k. Interested. Wow. Saying 300 k, confirm this business. I can pick up, right? Yes. So 300 k I have a very bad habit of [00:14:00] it's a very funny habit. So every time when I actually look at the big data, I will actually look at the id. So I actually do a D tip on the, ID find out how many duplicate cases are there on the id. So yes. So what? 300 K data? Only three customers.
[00:14:15] Andrew Liew Weida: Is it a B2B customer? Very large customer
[00:14:17] Jarrod: base. Therefore only, no, they were saying that no, it's a B2C and I, a general hundred K customer, we 300 K customer. And then you can do something about it. Then I should do a d B2C unique customer. And he wants to profile the entire nation with this
[00:14:35] Andrew Liew Weida: data. Wait, b2c three customer. Is it sell sell like Twitter to, there's only
[00:14:43] Jarrod: if that I will actually want cut a 1 billion, right? So . then, you see 300 k and then he say he wants to use this 200 K to prefer the entire nation on energy saving. Wow. Such a nobody And then, and not only three [00:15:00] customers, I can't do anything. Now
[00:15:01] Andrew Liew Weida: Do, you ask them how come or what could the reason be when they have this only data set, only have three double duplicates
[00:15:08] Jarrod: data.
[00:15:09] Jarrod: It's because it's the energy consumption usage of these three customers. And you know how their data is they, gimme a data dictionary. They're so proud that Data Dictionary has said that this is a majestic data dictionary. So I look at it okay, Voltage description is voltage, and description is, and right. All the third column on words I really give up already. That is current. The current in the variable name, the description is current. So I was actually asking him how am I going to understand what does the voltage used for? Is it for fan, is it for fridge or, is it for Acorn or whatever Is it, oh, voltage is voltage
[00:15:52] Jarrod: I can't do anything for them like that. .
[00:15:56] Andrew Liew Weida: I know. I think I had a very similar case for a [00:16:00] travel company. And they say, oh, we wanna build the best AI system. I say, okay. They got really a lot of data, let's say in one tete. But the, problem is that the data was all over the place. And then I say, okay how can I piece out the data together? What's the unique key? You must have some customer ID or some common id. Then, you know what they say because of this like privacy data act from different country. Then the, data engineer and some engineer they built with different ID key. There's no ID equivalent nobody create an ID to piece all the data together, expect me to be the encryption magic guide to all this data sector, to this data set.
[00:16:47] Jarrod: How, I'm gonna do that. I, dunno how you're gonna do that as well. I have no, I, okay, I'm, I, although I'm achieve data scientist, but then if you actually gimme this car situation, I also dunno what to do. I can only say good luck , [00:17:00] how about this? We look at just one data itself and then we try to actually solve something off with just a simple dashboard but then you just gimme one data without even ID to we don't even know that the ID comes from the same guy or not. I know what you mean. Yeah. . So yes, so listener, actually listeners, so you are hearing this, we data scientists actually are not magician. Yes. We, do have requirements from a data. If you, if your data fits into this kind of situation that we just talk about, it is time to actually speak to a data engineer, to help to clean up the data. We can't do things with tree customers to prefer the entire nation or entire World Tree customers to prefer the entire Not possible. Alright. As well ID key not we don't even know whether the customer is the same guy or not. There [00:18:00] is hitting 300 K inside your database. They're so risky to, come up with business strategy on just that itself. Too. And then the, other one actually talk about so, that is what, you can do with this data when your customer ID is not even there on my case. Only transactional information. And then you're telling me that you understand targeted information to customer, but send to who we can't
[00:18:32] Andrew Liew Weida: Yes. So don't you think that, I'm just thinking out loud because you mentioned about recommending starting data engineering. Companies to be before they begin the path to AI or digital transformation machine learning transformation. Yeah. But some of the C-suite people, like the ceo O and c CTO will say, Hey if we normally will hire data science or we outsource to a data science assignment because we don't even know what we [00:19:00] don't know, as they say we only hire data engineer. When the data scientist tell us all the shit that we need or the gaps, the areas of improvement, then we know what the hire data engineer what, do you have to say about that?
[00:19:13] Jarrod: That's fine. Then I can actually come in as an advisor. If you have money to spend, why not? Ah, we can look together on that. No, so if you wanna hire a data engineer, I can actually pinpoint the problems to data engineer because data scientists are not just expensive to hire, they're hard to find as well. That's true. That's true. Yeah. You can say there's a lot of graduates as a data s which is can bring them in as a data scientist, right? You are just actually killing, you're killing a young guy who is actually trying to be passionate or as a data
[00:19:43] Andrew Liew Weida: scientist talking about mental health, actually mental health, right? Yeah. Ah, no worries. Like all these junior guys can one, just hire them and then true at them. Then three months later, Hey, why the attrition is so
[00:19:55] Jarrod: higher? It's unfair that these young guys who are just really trying to progress in their [00:20:00] career, right? You need guys like us who actually seen the horror of itself in the database, and really travel through many data swan. Then you will actually understand. Okay, so this is the problem. We target the problem, we look at how we hire data engineer in to properly see how we can actually do things with your database while we data scientists the experience. One, look at your database and see what else we can come up with first as a low hanging fruit, maybe to actually see. Then we can actually build that on once we actually have a data engineer set up inside your company itself. Yeah. I
[00:20:39] Andrew Liew Weida: Don't, you think that there's also a new emerging trend called the data strategies work for veterans, like as a data scientist or senior veteran, but why they say that, okay we have a business problem before we get a data engineer. Can you guys give us a data strategies like you say that like this business problem requires a LE aggression, which is a numerical data scientist. [00:21:00] And then okay, this is the data, the output data unit. This is the input data unit. This is the normal question. Now this is a piece of work. Then you go and get a data engineer, go and do whatever the e lt. Do you think this is a, this data strategy work? I call data strategy. It's a emerging path of time to, to solve this bottleneck problem before they hire any junior data scientists or, Build a data engineering team and all thoughts.
[00:21:25] Andrew Liew Weida: Now normally the people ask me, go through the give the data make sense take the, business problem and then clean the data, build a data prototype, right? And then show and do show and tell and then put it in production.
[00:21:40] Andrew Liew Weida: Yeah. But then there will be companies I wanna get a sense of what is a data science work or data engineering work? Can you like it's like the the management consulting era, like the Mackenzie Boston Consulting Group and Bain before they do they tell me they'll [00:22:00] hire essential IBM m to build all this software and processes.
[00:22:03] Andrew Liew Weida: They'll get the, McKenzie guys and the business strategy guys to say, okay, this is your, business problem. This is the approach now, this is the, path now. Then you go and get the implementation guys to start hiring and building and operationalizing. So the, da, the data strategy G work is like, , you tell me the business problem.
[00:22:27] Andrew Liew Weida: I look at the existing nomenclature of your database. Or if you don't have database, then I'll tell you, okay, this the output that you want, this data of output. Let's say you wanna increase revenue. Do you have revenue data? If you wanna let's say boost your marketing. Do you have this marketing, have a customer id?
[00:22:45] Andrew Liew Weida: And there is these, all these factors are, I give you all the factors I tell which this factor what type of data, and then this is a piece of work. This is called data strategy.
[00:22:54] Jarrod: What, do you think of that? Yes, we can we, can work out something like that. That is[00:23:00] we look at the business problem, then we actually devise strategy possible one. But then it is actually the person who devis the strategy to actually continuing on the works, to actually look at the data to see whether it fits onto the strategy or not. , you can't have a separate, that is one group do the strategy and another group look at the data and then try to fit in the strategy.
[00:23:25] Andrew Liew Weida: The part and the testing part only comes when you have the data, right? Let's say. Yeah. But when you don't have the when, the client don't have the data, but they, want to figure out what they don't know. Before we, buy this. Technically speaking, I should be able to buy a house that is well designed. Every furniture, everything, electric, wiring, everything in but I, first of all, I do not know what I don't know. Should I buy a, a green wall or a blue wall? Can somebody just do a painting, a blueprint for me first? Yeah. Before they engaged us as the contractor means [00:24:00] we renovate for you. We drill this drill that do everything for you. We will ask you, do you have the budget and you want green color, blue color or not? If he doesn't know anything, how to get him to visualize to say, Hey is what I want. Okay. Now I let me get the data engineering first and then get you guys to help us handholding something like that. I dunno. What are your thoughts? .
[00:24:21] Jarrod: So you see this is actually a challenge of a data scientist as well. That is we usually start with the business problem and then we try to actually do a blueprint, right? But that's why I say the person that do the blueprint should actually go all the way down to the data to actually see whether he can enhance the blueprint along the way. Because you, can't actually do a blueprint as company A, right? Company A, done the blueprint rating, and then hire company B research, research a cheaper option, right? Say cost, cheaper option company B, it will actually do a, the fulfillment of [00:25:00] company, a blueprint that will actually be a horizon arena.
[00:25:03] Andrew Liew Weida: I would not say it's a cheaper option, rather it is once you have this, come back to me again. Because if they, don't have the data Yeah. They go and use the service it will take a much longer time. Yeah. But if they, if you tell them, Hey, okay, you pay me this amount. Here's the blueprint. You have the data come back for the full service.
[00:25:21] Jarrod: That's possible. But then that the company really must come back to, the person who suggests the blueprint. Because you see then, it is the, responsibility of the planner, of the blueprint to look at what is being collected and then see whether we can enhance the blueprint further.
[00:25:39] Andrew Liew Weida: I think the likelihood of the customer coming back to the guy who built the blueprint or built very high, because you when, you build the, let's say you give the, this is the dataset that you need, but you didn't tell them the algorithm. So they just get the dataset and they go to another data scientist. Data scientist, they don't have the previous contacts, right?
[00:25:58] Jarrod: Yeah, that's why you [00:26:00] see when I was actually at an e-commerce company, I was saying that, yeah, I can actually build a profiling model because this is actually what they want. They want you to know their customers, right? Yeah. So they say, we know our customers Mari status, we even know where they stay and all those things. It sounds very fairytale. You have everything of our customers from A to B, so I know that's from A to Z. Yeah, everything. So I know it's a red flag. So I say, okay, you have everything about the customers that can do profiling. Give it to me. So when I join, they have only agenda and H huh, . Yeah, I know. So, you see that's why yes, I design a blueprint and say that yes, we can profile a customer using certain model, but then when it comes to me and say that, oh, okay they only have gender and H so what can we do? So we have to enhance the blueprint, right? Like example data engineer or, maybe a poor young data scientist to actually fulfill the blueprint when the guy who [00:27:00] designed the blueprint has no contacts of the data. That would be very scary. .
[00:27:04] Andrew Liew Weida: I, would say it's very scary, but I think it happens a lot of times. It normally, it leads to high attrition rate, especially if they wanna build in-house hiring, because the previous guy, I mean in the name of hr, a lot of company like hr, the problem with Agile is that the documentation is very scared and a lot of the knowledge is actually embedded in the brain of the previous guy. So any new data scientist that just came out, data science manager. Hey, this machine learning 600,000 lines of code. Huh? Where's the documentation or the, predecessor just quit. Really? Ah wait, can, I'm sorry. I don't think you can, how do you solve this kind of problem, man?
[00:27:44] Jarrod: At least the court must have comments inside
[00:27:46] Jarrod: The courts have comments inside. Then it takes people like us who is actually went through many data, so went through many data codes even without the commands, is that the comments itself? We can [00:28:00] understand by running it, we can understand how, why do these commands are running like that, right? Roughly. Understand. But of course then we have a documentation. It'll be easier, right? But then Yeah, you're right. That is we, need we have this also this issue that this data scientist a quit and then leave a legacy and then the data science team, data scientist B, have to actually take over the work.
[00:28:27] Jarrod: It's also a very interesting legacy that needs to be taken over. I, had actually another example. Someone actually contacted me on LinkedIn and say Jared, I have a model that have totally no predictors inside the linear regress. all the models are actually unimportant. Why is this?
[00:28:49] Jarrod: So I never built model like that. Actually. It is actually surprising that all the business factors actually comes up as not important. Oh, . Then it is become [00:29:00] why go to C? Why go to constant? So plus error then or maybe, but that's all I gotta see. So, I was actually saying can you tell me your blueprint of it?
[00:29:09] Jarrod: So he say, we are actually using the travel data, right? To actually see what the customer want on the e-commerce company. It sounds very, doable because this is actually a blueprint that was designed but then was left by someone and then they are trying to fulfill it. And then I was actually asking, so who designed it that is travel and then to e-commerce.
[00:29:33] Jarrod: I didn't say the solution, but I was saying that if you pay me money or tell you what's, going on, yes. So, I was sitting around in the cafe. I was actually thinking why the hell that the business factors are all unimportant in the linear regression model. Then after thinking for a while, I was actually joking in my ceo, I say, you know what, it is just like asking McDonald's customers.
[00:29:57] Jarrod: Whether they are satisfied with [00:30:00] k
[00:30:00] Andrew Liew Weida: ffc, , . I, was You say that .
[00:30:05] Jarrod: It's very funny, right? The analogy nothing wrong with that. We, eat McDonald's early kfc, but otherwise looking for burger and otherwise looking for chicken. So you are looking Yeah. Are using travel data to actually predict e-commerce needs is Of course all the factors will be unimportant, right?
[00:30:25] Andrew Liew Weida: If, the target audience doesn't like you say, if that, if the McDonald lovers just want eat McDonald and they don't want give Cs.
[00:30:32] Jarrod: Yeah. One is looking for you, is looking for chicken. You . So, I was asking them this question. I didn't tell them the solution. I was asking this, question. Do you mind if I take over?
[00:30:45] Jarrod: They will recollect the data. Ah then, they stop talking to me and altogether they know what's going on. . Ah, I think they know what's convince it is a project that is actually going to be in failure. Basically. They don't, [00:31:00] I don't think they have much time left actually in this is the problem with designing blueprint.
[00:31:05] Jarrod: If you actually get a very seasoned data scientist to design a blueprint and that blueprint actually gives to newbie to work on, you are literally really asking for trouble. Yeah. I
[00:31:17] Andrew Liew Weida: think I talking about timeline, this is one of the things that I wanna talk about. Let's say for, C-suite guys, they want us to build ai, build machine learning, build transformation.
[00:31:26] Andrew Liew Weida: Where is it from? Scratch. I'm taking over. Like how do you respond to them when they say, Hey J, you can do it. You see it? Are you super good? You're super expert, you're superhero can you get this thing done in three months time? When, you know that, when you saw the size of the score or problem, this thing will be like a six months, 12 month, 18 months problem.
[00:31:46] Andrew Liew Weida: How do you respond? Or do you have any stories like that?
[00:31:49] Jarrod: I will tell them that if you want it to be in three months, there will be three months quality basically. So I will actually say one, 'em actually look at eight months quality instead. [00:32:00] So if you want three months is three months quality one eight months is months quality.
[00:32:03] Jarrod: I believe the eight months quality is much better. Yeah. So then, you see, someone actually told me before they want a machine learning model than done mix next second. I don't even, I haven't even seen the raw that he wanted. Next second. So next second. I had a PhD colleague. His face literally went white.
[00:32:24] Jarrod: I said, don't worry, let me handle the situation. So then what happened was that we had a meeting with Twitterer, right? So Twitterer wants to once asked to engage them. I say, yes, you can engage we can engage you. If you can give us machine learning model in next second, because you see. . My, the boss of that, client of mine is not interested in listening to an Asian guy.
[00:32:52] Jarrod: Oh, so I might as well let a Caucasian to actually list, talk to them. So, he always happen, I dunno why it [00:33:00] always happened that maybe they think that data scientist means he must be a Caucasian then can be a data scientist. So what happened is that I actually get a data guy and say that fine, you want us to engage, you can you must give us a machine learning model within the next second without even looking at the raw data.
[00:33:18] Jarrod: So he was saying, Jared, even our very experienced chief data scientist need at least seven months like that used to actually, or seven weeks to seven months like that. I can't remember the timeline to actually work out the machine learning. And that's actually a very trial format. How can you expect the kind of very weird request then that the client of mine who's sitting at right across the other end of paper say, Jared, I get what you're trying to do.
[00:33:45] Jarrod: I understand . So he dropped the idea of next second produce machine learning model without even looking at raw data. It's funny though weird, way that [00:34:00] maybe there are actually some, there are too much data conference that they attend. Yeah. And, then they saw that the data scientist presenter actually boom, See, this is actually my model. Boom, boom. This is my model. That's why I hate boom, data scientist . Boom, boom. That's my model. But it's not that, to do that you need to have a, to present preparation of your data first. Yes. Before you can even start that. Yeah. . I,
[00:34:28] Andrew Liew Weida: dunno. Maybe it could be another case where another sneak oil guard company is so then something and promise them next second.
[00:34:34] Andrew Liew Weida: I
[00:34:36] Jarrod: it's,
[00:34:37] Jarrod: very dangerous thing to actually say that next second. You actually come out with a . I don't even, I won't even trust the machine model .
[00:34:46] Andrew Liew Weida: This is this, that's the story of next. I have a story about there was this client that came to me and he say, Hey I paid millions of dollars to a brand consulting firm and they built this product, ah, they can [00:35:00] guarantee, return of investment, of whatever product that use this, diagnostic kit.
[00:35:09] Andrew Liew Weida: Then I was like, okay, then why are you looking me for you see? But after we, we paid this money and I get all the people to go outreach, marketing, outreach, to implement it of say 18 months. Really, I don't see any ROI on two things. , the product doesn't give ROI to my existing customer. ROI on my marketing.
[00:35:34] Andrew Liew Weida: Then I was like, okay, can you gimme a data? Then when I look at the data, I say, Hey, the, this is the claim. I say these are the factors that will guarantee this score and this score will guarantee increased revenue . And I was like, okay. Then I just basically look at that and then I ask that, Hey, what's the algorithm?
[00:35:50] Andrew Liew Weida: What's the method? They say, oh, they just built this product and then they just sell us this thing, close white package. They say, I don't even see the code. Then [00:36:00] how do I know? Can you get documentation? Oh, they say No, this is a well paper product. Then I say okay, can you go back to them?
[00:36:10] Andrew Liew Weida: Just give me the input output data, then just do my testing. Yeah. Then I just show them the result. You say that this input can, this to output doesn't work. Yeah. And then they kingdom me and say, can you rebuild this for me?
[00:36:29] Jarrod: A reverse engineer,
[00:36:31] Andrew Liew Weida: reverse engineer, where I don't even know the algorithm and you can't get it from your, and how do you respond to that?
[00:36:37] Andrew Liew Weida: I also wonder,
[00:36:38] Jarrod: Let's not doubt that the product actually doesn't work. it's, you have to speak with the person that is in charge of the product. He might have a certain vision of how the product should be used and how it should be used, right? But then if the person who used it wrongly and didn't come up the expected outcome, [00:37:00] it's not fair to you to be asking as a scapegoat or body back to actually say that, oh, because you did a shitty job of not proving that the model doesn't work, then it is not the business of the stakeholder.
[00:37:14] Jarrod: No it's, not like that. It's that's why I say that whoever that implement the machine learning model blueprint or the product, you should sit through the entire thing and tell the client that why is it working, why is it not working? And things like that. So for, example, my model itself, yes, I will tell my client that it is not a magic pew, right?
[00:37:34] Jarrod: If you don't, if you introduce new product, which is today introduce, you cannot actually expect it to have roi mix again, right? So you, today is a newly innovative product that is on your end, like a shoe, a new shoe, new pair shoe, right? New brand. You cannot expect it to have millions of dollar tomorrow, right?[00:38:00]
[00:38:01] Andrew Liew Weida: Hi guys. Thanks for listening to this podcast. In Part 4 with Jarrod, both are exchanging stories on dealing with weird customers. They also talk about the importance of managing the client’s expectations and scoping of the work alongside with the budget. Jarrod shared his view on the future of AI. He believes that Elon Musk is very likely to be right about the future of AI. He shared his favourite book on AI and tell a story of how his mentor helped him. If this is the first time you are tuning in. Remember to subscribe to this show. If you have subscribed to this show and love this. Please share it with your friends, family, and acquaintances. See you later and see you soon.