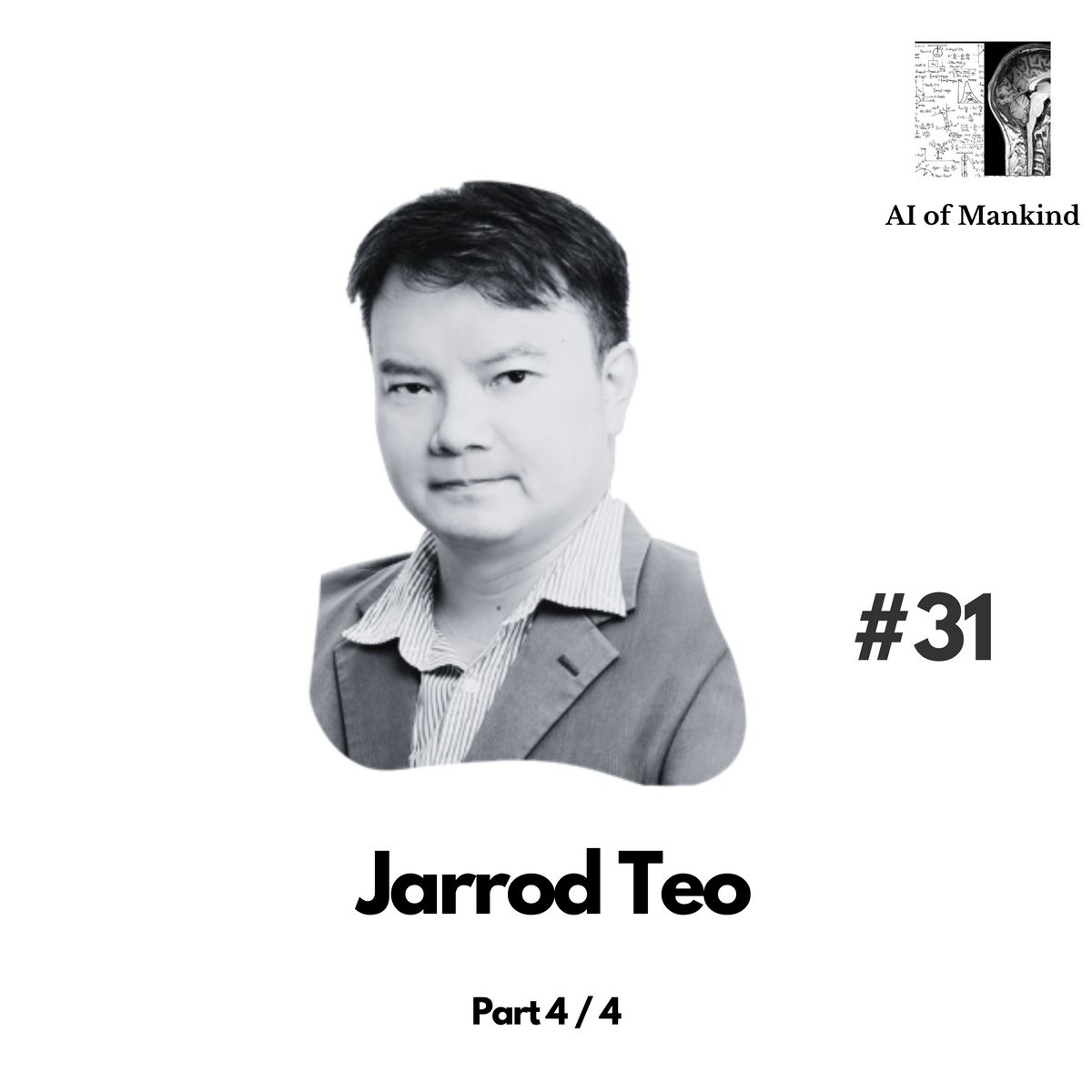
Trailer
Podcast with Jarrod Teo Part 4
Summary:
Jarrod and Andrew, discuss their experiences with strange customers and the importance of managing expectations and budgets. Jarrod also shares his thoughts on the future of AI and mentions a book on the topic that he enjoys, as well as a story about how his mentor helped him.
[00:00:00] Andrew Liew Weida: Hi, everyone. Welcome to the AI of mankind show where I share anything interesting about mankind. I'm your host for this season. My name is Andrew Liew. I work across four Continents and 12 international cities. Also, I work in tech startups across a range of roles from selling products, making customer happy, figuring out fundraising, making finance tick, building teams and developing sticky product. Apart from building startups. I've also worked in fortune 500 companies as a chief data scientist or technologist or people leader. You can call me Jack of all trades or master of learning. I hope to make this podcast show a great learning experience for us In each season, there is a series of interesting things where invite guests to share their views about their life and interests.
[00:01:09] Andrew Liew Weida: Now let the show begin.
[00:01:26] Andrew Liew Weida: In the previous episode, Jarrod and Andrew exchanged views on the difference between a data analyst and a data scientist, the difference between a technical data scientist and a client facing data scientist. Andrew shares a trend that companies are seeking data strategists to build data roadmap and data blueprint for companies and Jarrod mentioned the need that companies do not just stop at that stage but follow up closely to implement the roadmap and blueprint to avoid a translation gap. Both agreed that companies need to be patient with implementing data transformation as Jarrod share a funny story about Twitter wanting a machine learning model in the next second.
[00:01:59] Andrew Liew Weida: This episode continue the part 3 conversation with Jarrod and both are exchanging stories on dealing with weird customers. They also talk about the importance of managing the client’s expectations and scoping of the work alongside with the budget. Jarrod shared his view on the future of AI. He believes that Elon Musk is very likely to be right about the future of AI. He shared his favourite book on AI and tell a story of how his mentor helped him. Let's continue.
[00:02:25] Andrew Liew Weida: Then I was like, okay, can you gimme a data? Then when I look at the data, I say, Hey, the, this is the claim. I say these are the factors that will guarantee this score and this score will guarantee increased revenue by 99.9%. And I was like, okay. Then I just basically look at that and then I ask that, Hey, what's the algorithm?
[00:02:44] Andrew Liew Weida: What's the method? They say, oh, they just built this product and then they just sell us this thing, close white package. They say, I don't even see the code. Then how do I know? Can you get documentation? Oh, they say No, this is a well paper product. Then I say okay, can you go back to them?
[00:03:03] Andrew Liew Weida: Just give me the input output data, then just do my testing. Yeah. Then I just show them the result. You say that this input can, this to output doesn't work. Yeah. And then they kingdom me and say, can you rebuild this for me?
[00:03:21] Jarrod: A reverse engineer,
[00:03:23] Andrew Liew Weida: reverse engineer, where I don't even know the algorithm and you can't get it from your, and how do you respond to that?
[00:03:29] Andrew Liew Weida: I also wonder,
[00:03:31] Jarrod: You have to speak with the person that is in charge of the product. He might have a certain vision of how the product should be used and how it should be used, but then if the person who used it wrongly and didn't come up the expected outcome, it's not fair to you to be asking as a scapegoat or body back to actually say that, oh, because you did a shitty job of not proving that the model doesn't work, then it is not the business of the stakeholder.
[00:04:00] Jarrod: No it's, not like that. That's why I say that whoever that implement the machine learning model blueprint or the product, you should sit through the entire thing and tell the client that why is it working, why is it not working? And things like that. So for, example, my model itself, yes, I will tell my client that it is not a magic pew, right?
[00:04:19] Jarrod: If you don't, if you introduce new product, which is today introduce, you cannot actually expect it to have roi mix again, right? So you, today is a newly innovative product that is on your end, like a shoe, a new shoe, new pair shoe, right? New brand. You cannot expect it to have millions of dollar tomorrow, right?
[00:04:41] Jarrod: So, then if you want to actually sell this pair shoe to a customer, you want to sell it to someone, Is likely to buy a shoe, right? Yes. But then because this new product that is totally not in your DNA of the company marketing procedure, then you have to fall back on. So what are actually the face of your customer that is likely to actually buy an expensive product?
[00:05:06] Jarrod: Then you actually push these shoes accordingly under those underlying products that the, customer is likely to buy. Oh, this is a new product, and see whether it is a catch or not. Then slowly build out the brand of the product. So then you, it's actually the person who designed the model, designed the blueprint should actually sit with you to actually discuss about how he's actually expecting this productive function and how the output is going to be locked.
[00:05:35] Jarrod: How you going to measure the outcome as well, even if the person is not going to tell you what is inside the machine and model. No
[00:05:41] Andrew Liew Weida: What, happen if the previous vendor, sees you as a competitor and refuse to cooperate? Because knowingly that the client wants immediate results and after 18 months of like marketing campaign and using the model and the product
[00:05:59] Jarrod: Just say that. Just replace that model because after 18 months it doesn't show any result. It just means it's not really. Isn't it?
[00:06:07] Andrew Liew Weida: Let's say it actually happens that like, that, so I told the client, I did a report. I say, okay, after you did consultancy with me, this is the result.
[00:06:17] Andrew Liew Weida: Then he say, okay, now the second piece of it, can you rebuild the whole thing for me? Then I say that, okay, this is the budget. Let's say you need all the data, cloud, infrastructure, everything like, because all the existing facility, all the data and all the computing model and software is hosted at the vendor site and the vendor refuses to provide it.
[00:06:41] Andrew Liew Weida: So I say you wanna review everything from data to software engineering to apps, and to end you need at least half a million dollar. Then you say don't have half a million dollar, can you do it for hundred thousand dollars or like less than that?
[00:06:55] Jarrod: Not, possible. Because you see, someone actually told me before Jira, can you actually do an Amazon website with checkout, with whatever and then shipping or 300 over dollars as it not possible, right? Amazon website with checkout functionality with shipping and orders for $300. He say 150 more is gonna find expensive. So you see this is actually a bit of just really managing the expectation. We, really had to build, from what I see, if it doesn't show result in 18 months and the, vendor refused to hand over whatever that is needed it's time to review and then when it's time to review it, you have to actually pay you accordingly, isn't it?
[00:07:43] Andrew Liew Weida: Yes. I, think the challenge is, I think is a sun cost fell like the guy spent a few million dollars of a big brand and I won't say a nobody like us, but rather we, we've been through the shit and gone through a trenches and before them what is fair, wise, realistic lab. And then they second, So for that particular case, I literally was very professional. I say okay, may maybe once you have the sufficient resources, then we can revisit the conversation. And yeah. But like having along this line, like what is the advice that you, we can give to the data scientist that wants to be a front end data scientist or how to build the business a human or nuances with the business so that they
[00:08:25] Andrew Liew Weida: can understand the data better?
[00:08:27] Andrew Liew Weida: There is actually no one statement that I can actually gift that. We'll actually buy tomorrow. The person become the front end data centers. So you can see that entire journey actually takes years. You an analyst first, you understand the tax tech and all those things. You understand how to frame a business problem and even a simple univa why univa analysis is important because this is actually why p your data, right?
[00:08:55] Andrew Liew Weida: Yes. And analysis is also pie and parcel of data cleaning. So then along the way then you know how to run a department, and then that's where you're able to also, because you talk to a lot of client, then you're able to actually frame business questions accordingly. So it's not an say today I graduate, tomorrow I become a front end data scientist.
[00:09:17] Andrew Liew Weida: Not possible . Yeah. Because if you are trying to do that you are asking for trouble because there are actually entrepreneurs actually been drawn to jail, right? Because Yes, she says that man, yeah. A travel blood can actually measure everyone's information. Yeah. Yeah. So yeah, you can't just, it takes years to actually master this skew or become a front end data scientist else.
[00:09:49] Andrew Liew Weida: Really.
[00:09:51] Andrew Liew Weida: Yeah. Okay. Cool. And then next interesting question is what's your view on. ai. What's the current view and the future view of ai?
[00:10:01] Jarrod: The current view of AI is that a lot of companies are still collecting data and trying to actually make hit, and tails of it. So anyone who is able to actually do productization or maybe say even to run successful machine and model, they, they are actually on the slightly advantage portion.
[00:10:24] Jarrod: And the future view of ai, they could actually go more into ums of cybersecurity or protection n because it's so easy to actually get this information from the AI pipeline itself if the hacker is actually skewed so, then the, future of AI could be also about protection, number one, and also about you see more maybe the Ironman stores coming up Yeah. Yes. So I hope this won't happen more of unmet stores because I, still want to actually go to shopping more with someone talking to me . So I've actually been to Amman store around my neighborhood and then I've spoke to people who create ai, human. . Yeah. Yeah, I've actually seen that.
[00:11:19] Jarrod: So we might actually see more of this kind of when you actually have five pieces of data science coming together, you'll see this kind of am install doing recommendation to you as well. Yeah.
[00:11:33] Andrew Liew Weida: Yeah. And, so talking along that line how we mentioned about more protection, more cybersecurity in the future view it just reminds me about like I think a few years ago there was this Elon Musk and Jack Ma, they were having this debate about AI and the Jack Ma schools are thought he's optimistic.
[00:11:52] Andrew Liew Weida: He was a, that AI will do a lot of crazy things for the better of humanity. And then Elon Musk is oh no man, it's gonna AI is good. It will create a lot of good stuff, but if we don't manage well, we might become slave to the master of AI for use of weapons, weaponry or surveillance like in China.
[00:12:17] Andrew Liew Weida: What's the view? Who, who do you are more inclined to visit the Jack Ma school of. Or the Musk
[00:12:24] Jarrod: Personally don't like El Musk, but ? No. I will prefer to go with the Elon Musk of, because what he say is right, that is if we actually create a superior being that is able to go onto Archive Cloud to actually learn a skew and then replace us the next, again, it's not actually being very good to the human society as well.
[00:12:54] Jarrod: Yeah. So then we, are literally having this superior being replacing a lot of people and then this superior being that is the ai human, they might actually be thinking, say that we want human right because we are better than humans. Ah, then they'll find us slow because they actually can learn skills faster than anyone go club and then immediately pick up the skill, right?
[00:13:20] Jarrod: Yes. So then that actually is having a danger of an AI human actually having an equal rights as a human. And what do you think will happen next?
[00:13:34] Andrew Liew Weida: It sounds like the, Hollywood movie where was it Tom Smith the, iRobot like this character and that the robots suddenly have consci.
[00:13:46] Andrew Liew Weida: and they collectively wants to empower the AI robot trend versus the
[00:13:53] Jarrod: human trend. And I don't think this will happen in one or two years time, thankfully. We just actually make sure that to, actually just to inform some of our fellow data scientists, just to actually be very careful.
[00:14:10] Jarrod: AI is a tool. AI is actually good to assist human, but let's not try to actually build something that will take away privacy or to actually harm our presence as human as well. .
[00:14:21] Andrew Liew Weida: So you think that eventually AI is almost occur Like, money or electricity where you need to have a regulator authority to ensure that any company or any organization they use AI has some kind of fail safe mechanism or, black box mechanism.
[00:14:37] Andrew Liew Weida: If there's a circuit trip, you can kill it, right? I don't know. What do you
[00:14:41] Jarrod: think? As long as it doesn't actually. Post as actually a, track to totally replace humans, that kind of thing. Yes, there are actually robots actually been out there helping humans to make coffee.
[00:14:57] Jarrod: If you are actually at racial cafe, they have actually robot arm making coffee over there, which is very great, which is very good itself, but it's, it doesn't replace the, human, because the human are still working side by side with the robot itself. This is this an extremely good setup, right?
[00:15:12] Jarrod: But if you actually talk about a complete setup whereby yes, a men's store yes, is the, human has to be, don't need to be bad there to be there, right? But they have an ai human to actually talk to you. But ultimately the snacks and all those are still replaced by the human.
[00:15:30] Jarrod: The store is still managed by the human, so ultimately, that's why I say that AI should be actually a tool. It should help human, it should not replace human. It's not gonna
[00:15:40] Andrew Liew Weida: replace human. It just reminds me of the days when I was working for some of the airline companies and we always talk about like aircraft, like Boeing or Airbus.
[00:15:51] Andrew Liew Weida: Autopilot. Autopilot was being done 15 years ago. They've been using statistics to create a black box that type do that and June electronic computerized, but they still need pilot. because if there's no pilots, do you think passenger would there to sit on
[00:16:07] Jarrod: a plane? I am not. I am not very optimistic about this one.
[00:16:11] Jarrod: You see my my cleaning board is still hitting my leg glass. So Yeah. The cleaning bot is advanced video image AI or whatever but it's still hitting my leg. So what does this show ? So you wanna actually tell me that the plane is automated by non-human? That would be very scary.
[00:16:34] Andrew Liew Weida: Yeah. And so eventually the airlines decided, I think we still need to put some pilot and train them in the event that all this AI doesn't work.
[00:16:45] Jarrod: Yeah. Because we, know, we build machine learning model. Machine learning model cannot be a hundred percent accurate. Yeah. And
[00:16:55] Andrew Liew Weida: We,
[00:16:55] Andrew Liew Weida: know, the simple data driven or when the predictor and the actual data fits in as a brick.
[00:17:01] Jarrod: Yeah. We always have a break because the model that we built are based on the data that we give it up to today. Yes. But then we never know what will happen tomorrow or the next day. And then this new data that comes in when it doesn't actually fit into the the model, the machine learning model that we built, then how it, so you see, cannot have a hundred percent accurate model.
[00:17:26] Jarrod: Sure. Yeah. .
[00:17:28] Andrew Liew Weida: And so that's why there's that human taking over that aircraft. We still have ultimately this human, I also believe that human of high, all the entireties so far that they can still manage the creation, the aircraft, the weather, cause that's what they built for. Okay. Interesting.
[00:17:47] Andrew Liew Weida: What about the next interesting question is what's the view on the future of work? As there's small technology and companies are going into hybrid or remote work ai what's your view?
[00:18:00] Jarrod: We, can't stop companies who says that they want workers to come a hundred percent in the office, right?
[00:18:08] Jarrod: Yeah. And there are actually some works that really require humans to be in the office itself. Yes. Especially those machine types that require you can't actually install those machine in the house. It's just, instead the person needs to be in office . Yes. COVID actually tells us that we do not actually hold hands and do innovation in the office.
[00:18:32] Jarrod: This is true. Some jobs maybe say data scientist job, maybe we can have hybrid depends on whether the company culture actually allows a total work from home setup. Then, we can actually, of course it's really up to the company to. . Yeah. And talking about
[00:18:55] Andrew Liew Weida: that about culture what, do you think is a good culture to enable prospering data science work or operation in the organization?
[00:19:06] Andrew Liew Weida: Because you mentioned about the, junior data analyst before you eventually collect enough skills and nuance and knowledge to become a front end guy and even to become a data science partner where he can talk to business and solve and delegate he needs to learn to ask questions and even ask stupid questions, like to understand the data.
[00:19:24] Andrew Liew Weida: How, do enable such a culture to happen
[00:19:27] Jarrod: here? We have cultures that don't allow us to fail. Yeah. This is actually upset. Yeah. Some companies you, need to actually just they're not allowed to fail. You're not allowed to ask stupid question. We, just need the kind of environment which allows the new guy to ask questions without being fear of being actually in escorted or punish.
[00:19:55] Jarrod: We need that new guy to be able to experiment and, try out things. So for my team itself, I keep telling my team members that it is okay to fail so long as we are not delivering the product to the customer yet . So I, will tell them, just keep on trying. If you fail, tell us what way is. . Then if it's something that some other people in the team can help, like this is just a procurement.
[00:20:23] Jarrod: We need just a new product, new software to actually help you to work better. At least when you fail, you tell us, we can actually understand how to help. Of course, most of the time as data scientists, we know that if we fail in a machine learning model, our business stakeholder can help. The, most they can help is say, how is how you going?
[00:20:46] Jarrod: How is how things going? Is it actually fine? Whatever. Or maybe say, assign us to the right data engineer to actually work together. So then, we, data scientists needs to actually have a room to fill and a room to ask questions without being in fear of being punish, without, with when so that it's the same thing before the final product has, is actually been delivered to the client.
[00:21:13] Jarrod: It is okay to try. That's why it's called data science, right? Science is always a about experimenting and trial and error. Yeah.
[00:21:23] Andrew Liew Weida: Okay, great. Now the, what else does I'm thinking of asking about say that the interesting part about in, in, in the course of your career, what are some of the things that you wish when you were a fresh graduate or when you, wish you would've known when you were a junior?
[00:21:43] Andrew Liew Weida: Data. These are creatives for the, graduate
[00:21:47] Jarrod: staff. I wish that I can have someone to tell me what is actually the statistics books to read up? Yeah. Because the, time which I really pick up the skew is when I was in IBM s bss. And, that's actually where I pick up a lot of statistical books to start reading.
[00:22:08] Jarrod: And what I do is really literally pray and spray that is just pray and then just read up all kinds of statistical books. And then white paper, I'll read, I'll literally read seven white papers. And because I know that some of the white papers might need additional information to confirm whether it's actually that information is as it is.
[00:22:31] Jarrod: So I'll read seven of them and then because it's a new knowledge, then once I actually have all the white papers aligned with the thing that I'm interested to read up, I say, okay, in conclusion this is actually how the statistical methodology might work. Then I are tested on real data in order to see whether it is as it is.
[00:22:53] Jarrod: So, you see, I wish that someone can be there to tell me, oh, Jared, you need to actually read up. These basic stats first or jar, you need to actually then look at this models, what is what are these models used for and, things like that. But I don't have, what I have is literally, I don't have money to do master or PhD.
[00:23:17] Jarrod: So what I did is really to actually just read white papers of the white papers and my IBM statistical books are the statistical books. And then pick up a lot of things like ama pick up things like en Cova and Man Nova and all those things. And then, yeah, that's actually how I survive
[00:23:40] Jarrod: Oh, but
[00:23:40] Andrew Liew Weida: Having said that it's commendable. You, made up for all the deficit of formal education with informal education, which means that you, self-learn, you realize that hey, there's a need to be a video scientist. I need that depth of knowledge in terms of the statistical tool kids, right?
[00:23:59] Andrew Liew Weida: Yeah. There's a lot of times in any banks travel company for career, I consult for when, I was trying to guide and coach some of the juniors, they don't seems to be interested in the understanding of the statistics technique. They were more interested in the, okay, is this a package? Can I just.
[00:24:19] Andrew Liew Weida: Push in a gi big button, you just press the comic button and it runs . If you dunno what the intuition, behind or the idea behind this GitHub package or whatever package that you use, if something goes wrong, how do you know whether is it wrong or is it wrong because of the data? Or is it wrong because of the techniques that fits to the business problem and what the data manager is full?
[00:24:44] Andrew Liew Weida: Or what do you have to say
[00:24:46] Jarrod: about that? If we don't know how our models work, we don't know how to enhance it. This is actually the, a very straightforward thinking. If we don't know how the statistical analysis work, we don't know how to use it properly as well. So, if you don't want to learn, then think that it's actually just a package and then push out and then it's done, then you are no different from a tool and the tool is easily replaceable.
[00:25:12] Jarrod: Yeah. So if you want to actually be that guy who is, everyone will turn to and say that he can help the problem or he can actually do something about it, then you need to actually be able to actually say, oh, okay, because is this business objective? You have this data, then we should actually come by.
[00:25:31] Jarrod: This solution. Although initially I say that this is business objective, we use solution A, but because you actually have this kind of data we should use, right then. Then you can actually talk about this when you are able to understand how the model work. Because I always actually tell people models statist models or computer science models they are like U dances on French dining table.
[00:25:58] Jarrod: U issues have their own usage. If you don't know why data regression needs a dependent variable to be scale versus a logistic regression needs the dependent to be categorical. Then that's it, .
[00:26:15] Andrew Liew Weida: I understand. And then cause I, I use a different energy. I always like to use the, carpent entry.
[00:26:21] Andrew Liew Weida: Like you can't just use a hammer though, just because you have a hammer. Just every problem. You just hammer it. Some just need a flip screw driver to
[00:26:30] Jarrod: twist and turn right. Everything. Just use neural never.
[00:26:33] Andrew Liew Weida: Everything,
[00:26:34] Andrew Liew Weida: Neural network allow everything
[00:26:36] Jarrod: you can't just use neural network everything.
[00:26:39] Jarrod: I was actually I'm, actually not a big fan of neural network. But then the, ironic thing is that just actually a few months ago I was attending a data conference event. I met a director who actually came out of me and said, Jared you train me on neural network many years ago.
[00:27:01] Jarrod: I still remember, how you train me on neural network. Yeah. I don't like neural network, but But the reason why I don't really like neural network is that when you, when the business people wants to actually understand more about what is inside the neural network itself, we know that it's a hidden layer and it actually goes into a lot of technical explanation to a business owner who just wants to earn money, right?
[00:27:24] Jarrod: So if you actually explain things to them in decision three. , right? At least it's a is the tree is possible. I know you can see that. The profile is easier for him to relate. Then if the tree is something which you wanna enhance on, then you build it into a XG boost, right? After he understand the profile of the customer in, in, the sense you need to be layman enough to be a front end data scientist.
[00:27:54] Jarrod: Because if you actually think that everything is actually just pushed by a package and then it will run fine one day, when you actually explain things to the investor, he will not actually accept it, that, oh, because it's a package and then we just push it up, you'll run fine. But the investor is actually holding millions of dollar to invest in the company.
[00:28:16] Jarrod: He will not want to invest in a company like that.
[00:28:19] Andrew Liew Weida: I, think that's what they call the explainability, right? Whereby being able to explain how the model works to solve the business problem or whatever problem they're trying to solve. I think that's lacking in a lot of junior data scientists or junior data analyst that I see like, you say these days, everybody just tell me what's the package?
[00:28:40] Andrew Liew Weida: Just push it through. Oh, it was okay, I've done my job. They don't even bother.
[00:28:44] Jarrod: Is this the right this, is very scary we, then the person is as good as a package. Yes.
[00:28:51] Andrew Liew Weida: I, told them, Hey, the way you do it, how is, how you different from a data robot, right? These days you, heard of it, right? Just put a data inside and they turn out 1664 different models. And then, oh, this model has high accuracy. Okay, I push the package.
[00:29:05] Andrew Liew Weida: Go. Then when the stakeholders say, oh, the car accident or assembly front on the bank, then they cut the business guy come back to you, Hey, what happened? What happened then? Oh, Noah, is this the data robot law? 16 different packages, this package
[00:29:20] Jarrod: works. I just push it too. This is very scary on how things work like that.
[00:29:25] Jarrod: I will not want data scientist to, in my team to work like that. Honestly speaking, even my client engineer actually asked him, so you wanna actually use this as connection? Can you tell me why, you wanna use it? And why's actually advantage? Or what are the disadvantage that we might see? And things like that.
[00:29:43] Jarrod: And then they will explain, because you see it's a difference between people who wants to know more and people who just, want to go by. There, there will be a time where people just want, those want to go by. If they know, they suddenly say, oh, I want know more, then that will be different because then they'll actually advance in a way beyond just the package itself.
[00:30:07] Jarrod: They will actually go into the front end data science thinking. .
[00:30:13] Andrew Liew Weida: Yeah. I, feel that I'm already aligned with you. I think having the data scientist being able to understand like the limitation of the model, right? Yes. What works, what doesn't work can, we even do a hybrid, different type of models or do a few models and then understand how it works?
[00:30:31] Andrew Liew Weida: Then recommend to the client at least explain to the business user that, oh we have a few models. This one might not work very well in accuracy, but it can explain this one work very well in high accuracy, but we couldn't really explain all the factors because it's a newer network. There's so many underlying mechanics.
[00:30:52] Andrew Liew Weida: It's best to have a bit of a few, and then when things don't work, you know what to treat. I dunno. Yeah. Yeah. Cool. Okay. Like you have created so much value so let's ask another interesting question for the users or audience. What is your favorite application that you like to use on a daily
[00:31:13] Jarrod: basis?
[00:31:14] Jarrod: Application is in software or statist model. Yeah.
[00:31:17] Andrew Liew Weida: Oh. Could be okay. What's the favorite software or what's your favorite daily application? Digital application, because the audience might wanna try something.
[00:31:29] Jarrod: There's no favorite application of software because I've actually been to multiple research house.
[00:31:35] Jarrod: If you read my profile on Lincoln, you know that I'm from new and I'm from beacon Consulting. We, service different client, even IBM sbss. We service different client and then they have an environment that we need to fit into. So it doesn't mean that we, know s spss statistics today, we don't need to know by tomorrow, or we need to actually know by today.
[00:31:58] Jarrod: We don't need to know our tomorrow. It's not like that. Whatever works basically then.
[00:32:03] Andrew Liew Weida: Yeah. But then, but let's say if the client were to say, Hey, Gerald, I give you all the money and whatever tools you want. What is your favorite that you, would use if given a
[00:32:13] Jarrod and Andrew : choice?
[00:32:15] Jarrod: Again, there's no framework as well because I might actually use as business statistics to actually run some data checking.
[00:32:24] Jarrod: Then I'll go into Python to actually use my three libraries. Then I'll go into alters maybe to actually run the pipeline. But then Alteris itself, I might actually use modeler. It depends. And then visualization. I'll use Power bi, right? Of course I can use, but he, can say, I, I just leave it to you.
[00:32:45] Jarrod: If you say there's just one application that will use in a data science project is not possible because you can't just say, oh, I just use Python all the way. Because ultimately you need to visualize. So you, need to visualize the power bi, right? You can use our. , but then ultimately is still boil down to what is the business objective you want to solve.
[00:33:09] Jarrod: What is the data that the person have? Then the, people say, oh I learned Python. I'm a data scientist, but I can tell you Python or are, is this actually a small D of a data scientist? Yes. Yes. We need to call, we need SQ as well to call, to extract the data, but then we, this we, need the codes to actually run, but ultimately we, need to solve the district problem.
[00:33:39] Jarrod: Yes. Yeah.
[00:33:40] Andrew Liew Weida: Okay. Okay. Maybe another interesting question is what's your favorite book that you read or reread or you recommend to the audience? .
[00:33:50] Jarrod: I don't really read books these days. ,
[00:33:53] Andrew Liew Weida: you read a lot of statistics books. What's your favorite statistics books.
[00:33:58] Jarrod: Oh, okay. I can remember because I'm, very busy to read books these days, but what in the past I'd really like to read is actually this book by Prof Ko.
[00:34:11] Jarrod: He's actually one of my mentor. Oh, okay. And then he, actually wrote this book that is on application of data science. I don't have the title of hand, but he's the one that actually talk about how to use the different model in tourism in he actually shows the project and then how he the thought process and orders and how he got the output.
[00:34:37] Jarrod: And then how to read the output is actually a very good book. Honestly speaking, if you actually have books like that, which actually shows you the thought process, the application of the model, and how it comes to a conclusion to a business objective is actually a, very nice book to read.
[00:34:54] Andrew Liew Weida: So you would recommend this professor Hawk Chai, right?
[00:34:58] Jarrod: Ko Chai, actually, professor Ko CHAI's book written by him is actually a very good book. Yeah. Okay. Let me see whether I can have the data offhand, eh ah, yes.
[00:35:09] Andrew Liew Weida: Of Ko Chai that I can put it on the, podcast cards and for people to check in.
[00:35:15] Andrew Liew Weida: Check it out
[00:35:16] Jarrod: The I hope you will be up . No worries. Let's see. He's he's actually very, he's kinda old now. . Oh, old is he now? Yeah. I've never seen him book like some time. This is he's quite old now. So basically he has this book that is data mining Application for Small and Medium Enterprise.
[00:35:38] Jarrod: This book is actually very good. Yeah. Is it a
[00:35:41] Andrew Liew Weida: very old book or It's a very recent, is it in the 1990s or 2000?
[00:35:46] Jarrod: Not in the 1990s, but you, if I can share you the link. Yep. How can I show
[00:35:54] Andrew Liew Weida: you the link in the message? You see it, the bottom right corner. There's this little chat with everyone.
[00:36:01] Jarrod: Chat with everyone?
[00:36:03] Andrew Liew Weida: Yeah.
[00:36:04] Jarrod: Okay, cool. Is this book you click on the link, you should be able actually see a, page. We actually shows learn at lunch. Or do you think on Oh yes, ma'am. Lemme have data mining application for small and medium enterprise last time. There's no data science. We actually called data science, data mining.
[00:36:27] Jarrod: Ah, okay. Okay. So Proco is actually the one that is in one, the screenshot. He's actually on the. This spectacle, high spectacle gentleman,
[00:36:41] Andrew Liew Weida: he's the guy that wear spectacles or didn't wear spectacle, didn't wear spectacle . Okay. So he's the younger looking one. .
[00:36:48] Jarrod: Yeah. So this one. So that's, actually one my mentor.
[00:36:51] Jarrod: He's a very powerful data scientist.
[00:36:54] Andrew Liew Weida: Yeah. Powerful in what sense? I think he's, very about Mickey, making it easy to understand.
[00:36:59] Jarrod: Very,
[00:36:59] Jarrod: knowledgeable. His book is very easy to understand as well. Yeah. Yeah. I think one of
[00:37:06] Andrew Liew Weida: The,
[00:37:07] Andrew Liew Weida: rescues that data scientists should have is the ability to communicate in people that can easily understand it as in cross translation manager talking to data scientists or talking to business guys.
[00:37:19] Jarrod: Yeah. Yeah. So see, this is actually also, that's why I emphasize if you wanna actually become a front end to present to investors, you cannot speak jargons. Yes, I agree. Any other questions?
[00:37:33] Andrew Liew Weida: Yes. Like to the audience out there, because you've given them so much what is it you ask what is it that you want to ask the audience who's listening to this podcast to be able to participate or support you given that you have given them so much who's listening
[00:37:49] Jarrod: to this?
[00:37:50] Jarrod: They can actually connect me on LinkedIn if need. I can actually offer data science advisors or maybe say to sell my Innovation DSS customer observer. I'm actually from direct sourcing solution. Of course it'll be better if we actually go on video version so that I can actually show how how does actually the DSS customer observer slightly works.
[00:38:17] Jarrod: And then what is the result that it brings in the past? Then, I think it makes sense to actually, if they want to actually communicate with me directly and ask for a demo, I'm happy as well.
[00:38:30] Andrew Liew Weida: Tell me more about this customer observation. Is it a
[00:38:34] Jarrod: product or a service? Tss? Customer observer is a product that I design myself.
[00:38:39] Jarrod: So it's a switch or product, and then it comes to tss customer tss, power profiler, tss, next product purchase, main genre and sub channel. And he has many other functional. And so this
[00:38:54] Andrew Liew Weida: product, is it is it across the function or is it for marketing or for finance, or what is this
[00:39:01] Jarrod: for? Any part?
[00:39:03] Jarrod: This one at the moment is actually for retail and e-commerce. So it's basically to help companies to make more money. Because they, will be able to actually understand their customer better and do planning business strategy and allows them to actually customer the right time.
[00:39:20] Andrew Liew Weida: Okay, great.
[00:39:21] Andrew Liew Weida: Yeah. So, the audience out there was listening to this Gerald has a wonderful product called dss Customer Observer. It's specifically to help customers, especially in the retail and e-commerce to increase revenue or increase sales
[00:39:35] Jarrod: for people who are actually in banking or insurance telco or maybe say mobile.
[00:39:42] Jarrod: Mobile phones. I, can do that dss customer observer as well. If you are in Coin exchange, I can actually do that as well. Coin
[00:39:51] Andrew Liew Weida: what you mean crypto exchange or what?
[00:39:52] Jarrod: So Coin, we once want to actually do one project for a coin exchange company. So it's like a stock exchange market, so it's Coin Exchange company.
[00:40:02] Jarrod: If people wants to do that in for coins we can do that as well. There is to find the right customer to sell the right coins to edit at the moment. I know it's not very good to talk about coins, but
[00:40:13] Andrew Liew Weida: you said FTX experience, right? I guess so. Yeah, that's right.
[00:40:17] Jarrod: Yeah. But we can actually help companies to actually find the right customer to sell the right product too. Even if you are in the banking or maybe it's insurance company, we did that last time. Yeah.
[00:40:29] Andrew Liew Weida: Cool. Yeah, that's the end of the podcast.
[00:40:33] Andrew Liew Weida: Hi guys. Thanks for listening to this podcast. If this is the first time you are tuning in. Remember to subscribe to this show. If you have subscribed to this show and love this. Please share it with your friends, family, and acquaintances. See you later and see you soon.